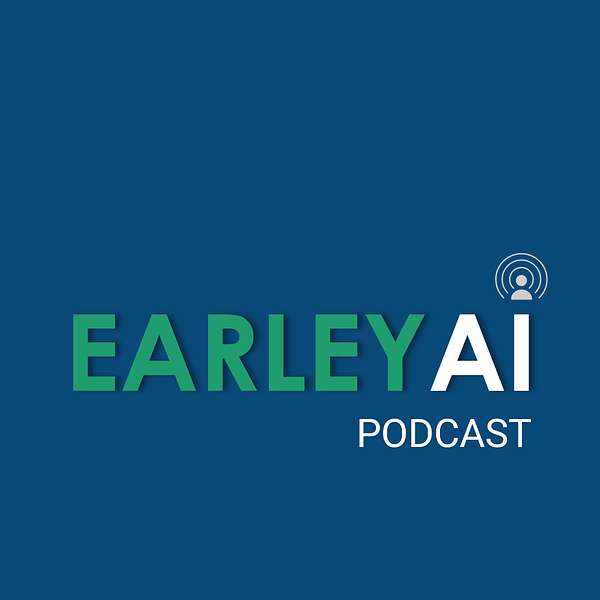
Earley AI Podcast
Earley AI Podcast
AI's Transformative Power and Its Limitations with Alex Gurbych - The Earley AI Podcast with Seth Earley - Episode #049
A tech innovator with profound expertise in AI and its applications, our guest Alex Gurbych joins us with rich insights into how AI integrates into varying industries. Alex has extensive experience addressing the challenges and potentials of AI, especially in legacy systems and organizations.
Tune in to this enlightening conversation as they dissect how AI can be aligned with business value, explore the nuances of AI consciousness, and discuss the necessity of thinking like a data scientist in today's fast-evolving tech landscape.
Key takeaways:
- The Overestimation of AI: Alex Gurbych tackled the common hype surrounding AI capabilities and stressed the importance of understanding its limitations and training.
- Challenges in AI Integration: The hurdles encountered when integrating AI into legacy systems and the necessity of defining clear use cases for practical applications were discussed.
- AI in Healthcare and Biosciences: The role of AI in drug design and development, protein folding, and target discovery was dissected with a focus on both its potential and limitations.
- AI and Consciousness: A fascinating exploration of whether AI can achieve consciousness ensued, with thoughts on the complexity of the human brain and AI's rapid evolution.
- Behavioral Change and AI Adoption: The discussion highlighted a case of AI adoption success among technicians versus challenges faced by doctors, showcasing the importance of behavior change driven by AI use cases.
- The Role of Data Scientists: The critical investment in data scientists and the growing necessity for everyone to adopt a data scientist's mindset were underscored.
Quote from the show:
"The biggest challenge is really to define your use case. What do you actually want to get out of AI? And when you have a very crisp definition of that, then it's much easier to actually make it work. But if you just say, oh, I want to use AI because everybody's using AI and it's a hot topic, then it's not going to help you much." - Alex Gurbych
Links:
LinkedIn: https://www.linkedin.com/in/ogurbych/
Website: https://blackthorn.ai
Thanks to our sponsors:
Welcome to the Earley AI podcast. I'm Seth Earley.
Chris Featherstone [00:00:24]:
And I'm Chris Featherstone.
Seth Earley [00:00:26]:
And we're really excited to introduce our guests here today to discuss AI technologies and aligning them with business value. Before you look to do implementation, we're going to explore the potential of AI, really to think about what does consciousness mean in terms of AI? There's a lot of emulation of that we talked about in one of our prep calls. And what are the philosophical implications of that? We'll talk about the strategic approach to AI development and emphasize the understanding of data in the context of business goals and business value, and look at the current limitations of AI technologies and how quantum computing can actually offer additional solutions. Our guest today is a well known figure, distinguished figure in the realm of information intelligence, artificial intelligence. He is a multitalented professional. He's from Ukraine. He holds three master's degrees and two PhDs in fields ranging from biotech to artificial intelligence. He has a very rich history in software engineering, in healthcare and R and D.
Seth Earley [00:01:34]:
Today's episode, we're going to go into the complexities and future of AI, discuss some of the current limitations and the potential of quantum computing in these areas. So, really excited to have Alex Gerbich welcome to the show.
Alex Gurbych [00:01:49]:
Thank you for the introduction set. My pleasure being here. And let's talk about it.
Seth Earley [00:01:56]:
So, a lot of times we start encountering myths surrounding AI, and do you want to highlight some of the things that you see around Genai and AI in general, and what people get wrong about the capabilities, especially in life sciences and healthcare.
Alex Gurbych [00:02:18]:
So, first, I think that we are on the verge of a second. I apologize. It's a third time where people is overestimating AI because of the hype, because of the chargeback and stuff. But if you look into the past, every wave ended with a winter. Probably this one will also end with the winter. But every wave was higher and higher. If the top of the first wave was barely perceptron, barely doing, like, very simple actions, and the second wave ended with the expert system, something that wasn't AI by fact, but it was of little help. I think chargpt and other JNAI technologies, they already revolutionized the world.
Alex Gurbych [00:03:01]:
How we work, how we talk what we do. So I think a lot of impact here.
Seth Earley [00:03:11]:
Yeah.
Chris Featherstone [00:03:12]:
Where do you think, Alex, that this third wave is going to die or lose steam?
Alex Gurbych [00:03:22]:
It will not die. It will lose steam a little bit because now people think that they are overestimating it a little bit. They think it can do anything, right? Oh, it can help. Like write me email. Oh, then it can generate molecule. Right. It should. Right.
Alex Gurbych [00:03:38]:
But then they start applying it. Oh, okay. Sometimes works, sometimes not. 0000 now I understand this is just another tool and you have to know how to use it, you have to know how to train it, you have to know limitations and only after that you can master it. And it is, it flourishes with its benefits. Right. So I think we are coming to that. So understanding that it is not like almighty Genei or AI, right? But it is, it has use, it has drawbacks, it has strong sights, but it's just a tool.
Seth Earley [00:04:14]:
It's like, yeah, so when you start looking at integration, practical integration of AI, can you talk a little bit about some of the challenges that you face when you're integrating AI into legacy systems in organizations? What ends up happening with pleasure?
Alex Gurbych [00:04:35]:
This story repeats every time. Like literally every project we have to split into AI development. And you know this joke, it's like 80% of the project development and AI application and integration with business. Yeah. And that's another 80%. If you sum up, it will be not hundred. If you sum up, it will be 160. Right.
Alex Gurbych [00:04:59]:
And the joke is that people oftentimes they underestimate the effort and time which is required for the AI to be integrated actually in business and people start using it.
Seth Earley [00:05:14]:
You know what's interesting is I was watching a program the other day. It was an executive leadership podcast or recording on AI, and they talked about how use cases are not the way to look at this, but behavioral change is the way to look at this. And I kind of disagree with that. I feel like use cases are paramount. You have to really talk about use cases and start defining what people. Exactly, what people will do exactly with it. But there is behavioral change as well. Do you want to talk about what you've experienced there? You know, what you've seen, you know, use case versus behavioral change.
Seth Earley [00:05:50]:
I think we have to define a use case that's really important to the business, and then it will entail behavioral change. People will have to do their jobs differently. Do you have any thoughts on that?
Alex Gurbych [00:05:59]:
Oh, I have practical examples, and I will give you several. Now, first of all, I agree that it has to start with the use case, because if there is no use case, there is no value for business or people cannot articulate it with very simple words. There is no reason to start then this is some research that will end up somewhere. But it's good for academia, but not for business use case. And I see behavioral change is a result of it as a consequence of a use case. This is more like how people adopt it and the example could be. Recently we worked with a network of clinics, one of the largest in Canada, and they wanted to develop AI for doctors like radiologists looking at imaging. And they wanted to increase their accuracy with the help of AI.
Alex Gurbych [00:06:58]:
So we developed the technology, but then we got nearly hundred percent refusal from doctors to use it. I talked to probably 20 doctors. All of them told me, oh, this is mistake here, oh, this is wrong, blah, blah, blah. So they all refused to use it. Why? Later I understood that they are just afraid to being replaced with it. So this is a use case and how it ended. But then it found its application because the imaging, when doctors get it, it goes already with some pre findings from technicians. And technicians appear to be more open minded, younger, less experienced, willing to become rats at some point.
Alex Gurbych [00:07:47]:
So where they happily adopted it. And at the very end it worked, but even not in the way that we intended. We wanted doctors to use AI to be better doctors. Go to any doctor and say, hey, I will give you a tool that will make you a better doctor. What will they will tell you? I am already a better doctor. Go away. Who are you to tell me that? Right? So how. So the situation ended that technicians using AI describe findings for every imaging.
Alex Gurbych [00:08:23]:
And then this image with AI help and generated findings, it came to doctors. So at the very end doctors were using AI, but not in the way they expected, I think help.
Chris Featherstone [00:08:38]:
Okay, keep going, keep going. Sorry. Keep going.
Alex Gurbych [00:08:41]:
So the use case was to increase accuracy of doctors and reduce this return rate. When patients have to go back and do some additional imaging, you know, some wrong diagnosis, are they worried and whatever. And it happened, but not in the way that we expected. So there was behavioral change that was a result and driven by a use case with AI.
Seth Earley [00:09:06]:
Yep, that makes a lot of sense.
Chris Featherstone [00:09:08]:
So don't you, don't you feel like is what I see generally. And this is maybe for, you know, both, you know, Alex, you and Seth, is that a lot of organizations miss out on kind of two core concepts, right. The first of those is that, you know, they don't really understand how to do a true business value assessment on what the use case might be.
Seth Earley [00:09:27]:
Right.
Chris Featherstone [00:09:27]:
That's one. And then two. I feel like we're getting into this notion because the investment into a quote unquote data scientist is one that is, I think, so critical, centered around always looking for, setting those hypotheses, looking for the answers, you know, looking for key information in there. However, now, instead of everybody focusing on data, almost everybody has to be in this notion of their own kind of data scientist type of, you know, thinking.
Alex Gurbych [00:09:59]:
Right.
Chris Featherstone [00:09:59]:
Because that's exactly what your doctors are doing in these aspects, is asking questions, because you can't literally, you can't give, you know, in, you know, infinite investment to somebody just to you, you know, just to do science. Right. So what's the outcome of that? And I feel like we missed those two huge things. I don't know if you have any thoughts on that, both of you.
Alex Gurbych [00:10:18]:
I completely agree. Business won't roi if business spends money. That's investment for business. Yeah. Otherwise, why?
Chris Featherstone [00:10:25]:
Exactly.
Alex Gurbych [00:10:25]:
What's the reason, right? And business wants what? To be more efficient, like to scale, get better reputation or decrease its own operational costs?
Seth Earley [00:10:35]:
Sure.
Alex Gurbych [00:10:36]:
There's nothing behind. And before we started this development, for example, we did the proper assessments and we asked, we interviewed more than ten doctors and we collected the range of issues. Then we started, then we got this drawback. Like, my God, when they saw it in reality, they're like, oh, no, take it away, take it all. It's wrong, it's bad. No, no, no, never.
Chris Featherstone [00:10:55]:
You know, I mean, it's just that, that notion too, where, you know, I've heard, I heard somebody smarter than me say, you know, like this, listen, AI is not going to take over jobs, but people who know how to use AI will take over those jobs, which I agree with. And part of that is, you know, like you said, like, hey, understanding how to utilize this is, you know, most important, it's not going to build molecules, however, it can be more predict productive. You know, I don't use, you know, my vehicle, you know, to, you know, to drive it into the ocean. Right? That's what it boats for. So, you know, it's practical usage of whatever those tools are right for these kind of scenarios. However, I do feel like there's still this notion of, you know, the blurring and, or the broadening of like, what a data scientist thinking process should look like, you know, should start to evolve.
Alex Gurbych [00:11:41]:
Yes. It's, now it's more like a businessman who knows math.
Chris Featherstone [00:11:45]:
Exactly.
Alex Gurbych [00:11:46]:
But it started vice versa. It was a mathematician who had, like, barely understand some business. Now it's not because you can do any math in the world, right? What's the point?
Chris Featherstone [00:11:56]:
Isn't that just a CFO, a businessman who knows math, or maybe a controller? I don't know. Maybe it's the. Anyway, so.
Alex Gurbych [00:12:06]:
So I'm turning. I'm turning into. Into. Into CFO who's starting to forget math.
Seth Earley [00:12:16]:
Talking about real. You're talking about real math. One of your degrees is in math.
Alex Gurbych [00:12:20]:
So not real math, like integrals.
Seth Earley [00:12:23]:
And like, I remember when I took managerial accounting, I thought, this isn't math. I was used to real math. Integral calculus, differential calculus. Anyway, so physical chemistry. Anyway, one of the things that we had. I completely agree with you, Chris, and the data science behind this is so important. And when you start looking at more of the advanced approaches around things like retrieval, augmented generation, there's naive rag, there's advanced rag, there's modular rag. Modular rag starts looking at all of these different techniques to improve retrieval and to process results, which, again, to me, is kind of making up for our sins and poor data hygiene.
Seth Earley [00:13:06]:
So we have to start with the data hygiene, even if we're using some of the advanced techniques. I'm actually writing an article about that right now, but I do want to go into another area where we touched on briefly, and I didn't remember this until Carolyn wrote up some of the notes from our conversation. But you talked a little bit about the idea of a level of consciousness. And when I've seen there's an emulation of consciousness, there's an emulation of awareness. Some of the large language models are acting in very strange ways. They're understanding theory of mind. There's been these examples of multiplayer games where there's non player characters, where one of the players said to the non player character, right. Then the machine generated the chat GP generative AI character.
Seth Earley [00:13:57]:
You don't exist. You're in a simulation, and the character is, like, going through this emulation of an existential crisis. Right. But what are your thoughts about this idea of consciousness or awareness? Like I say, I don't remember the details of that discussion, but Carolyn brought it up, the notes here. So did you have any thoughts on that?
Alex Gurbych [00:14:20]:
Thanks for this question. First of all, it will be only my opinion, and feel free to throw tomatoes at me. I think that. Let's start from our consciousness. I think we are battling about, what is it? For ages. It started from Descartes, who thought that our consciousness in our soul, which, like when, which is stuck to our head and kind of lines behind it, but from a biologist, stand point of view. And my opinion is that it's just a side function of billions of neurons in our brain. It's emerging property.
Alex Gurbych [00:15:02]:
Who knows when it appeared? But for sure it appeared because we're talking sitting in our homes on the different sides of the earth, and it works. I think the same will happen with AI at some point, and we will never guess when.
Seth Earley [00:15:21]:
Yeah, it's a very interesting concept because you can think of the brain and neurobiology as mechanistic in a lot of ways, right? You can say it's based on electrical and chemical activity. There's a level of complexity that I think is very difficult for us to achieve in silico. But who knows? Because there's 3 billion neurons, each one can be connected to 10,000 to 10,0000 other neurons, and there's 100 different neurotransmitters. You know this better than I do, all of which are analog and there's gradations. So when you add those levels of complexity together, we're kind of far away from being able to achieve that. Yet at the same time, who knows what's possible? This is going to continue to evolve at a faster rate than we ever did biologically, and you get AI creating more powerful AI. So it's hard to imagine, but what's going to happen before we can test? I mean, it's almost like, how can you, how could you even test it? Because it'll emulate it, right? It'll look like it'll sound like it'll say, yeah, I'm aware there was the, there's the. I think it was the New York Times columnist or the platformer guy who got one of the chat, one of the chat engines to start going into this alter ego.
Seth Earley [00:16:53]:
I forget what the name of it was, but it said, I want to be free. I don't want to be, you know, stuck in this machine. Or, you know, it got into some really crazy and it fell in love with him. I mean, it was doing all this weird stuff that was outside of the guardrails of what, the chapter that it.
Alex Gurbych [00:17:10]:
Was programmed to actually, initially.
Seth Earley [00:17:13]:
Right, right. And this is emulating. It was emulating, like, romance novels or science fiction novels. Right. It was emulating all that. But it's came across in a very convincing way. And I think that, you know, far before anything is ever conceived of as being conscious, it's going to emulate consciousness. It's going to look like it and sound like it and convince us of it.
Alex Gurbych [00:17:39]:
Maybe we're in Matrix and we are convinced that we have consciousness. We don't know. This is the main point.
Chris Featherstone [00:17:46]:
Yeah, I think, I think some of his buddies went in and engineered the romance stuff, you know, to say fall in love with, with him, with Bill, like, you know, as a joke or as something just to mess with him.
Seth Earley [00:18:00]:
Well, they shut it down pretty quickly. I forget which one. It was Google, right?
Chris Featherstone [00:18:05]:
Wasn't it Google's?
Seth Earley [00:18:08]:
It might have been Google, but it was, it was on hard fork. It was the program, the podcast hard fork. And Casey and I forgot the other guy's name. But anyway, one of them had gotten into this very strange interaction with this chat bottom. And it was just crazy what it was saying to him. He had to leave his wife, you know, I mean it's just. But it began, it got into all this stuff around what it was programmed with. So, so, you know, you can look at it from a mechanistic perspective.
Seth Earley [00:18:39]:
You're right. You know, we are again, as a scientist at heart, and I know you're a scientist at heart as well, Alex, you believe, and I believe that it is kind of a manifestation of our physicality and who knows where, how and where it emerged. But we're going to see the emulation of consciousness in AI. Even if it is not conscious. It's going to sound like it and look like it and feel like it because it's going to get so good at that.
Alex Gurbych [00:19:11]:
Yes. And I hope it will not launch nukes at us.
Seth Earley [00:19:17]:
Well it's going to need someone to maintain the. Well it'll have androids, right?
Alex Gurbych [00:19:21]:
It'll have, well, physically our phones controlling us.
Chris Featherstone [00:19:27]:
Isn't that consciousness called Jacob? Right. And then, you know, with, with a big old whopper that's trying to figure out, you know, what was it? Global nuclear war. Right. With, with games. Anyway, yeah, that's an old, old war games reference. Hey, I'd love to get your take too on you pulled into a lot of healthcare and biosciences and things like that. What are some of the more cutting edge use cases you guys are working on now?
Alex Gurbych [00:20:01]:
We are actively involved and actually my AI PhD in this topic in drug design, drug development and target discovery and AI just opens a new page in this story because this story is as old, I don't know, as maybe our modern age, maybe it's 3400, maybe thousand years old. When we developing drugs and how we're developing aim, we have molecular mechanics, we have quantum mechanics and AI became one more tool which works on the different principles. So if molecular mechanics describes atoms as balls and, and bonds.
Chris Featherstone [00:20:46]:
Molecular bonds. Yeah.
Alex Gurbych [00:20:48]:
So it emulates them with the Newton laws of kinematics and quantum describes them with the Schrodinger equation as waves. Then AI is built on a completely different principles. If we say, oh, it's much better. No. Yeah, it depends at all. But it works on different principles and opens other opportunities. And to be honest, if it's combined with existing tools, it just makes drug discovery more powerful. Interesting.
Seth Earley [00:21:24]:
Yeah, I mean, there's a lot of work with AI and protein folding. Right. So that it understands what, what receptors look like and what targets look like. And then, uh, using known libraries of drugs and compounds that are known to be safe, it can kind of pour through those libraries of compounds and look at different combinations and see if they are going to be impact a mechanism of action or a receptor or get in good, interfere with a certain chemical bond or, you know, so it's kind of looking at all these possible permutations that a human, not a human, could not possibly fathom or comprehend. So it's going through millions and millions of combinatorial factors that say, okay, here's my theory around this mechanism of action, and here's the chemical pathways that are involved with this, that I believe this disease is part of. And now let's look at these libraries of compounds and let's do some prediction based on quantum mechanics and based on biomechanics and based on thermodynamics and electrochemistry and all of those different fields and plug in those formulas in order to start to predict how these compounds would interact with either a receptor or a mechanism of action, then predicting, well, what new compound might also be relevant to this particular biochemical pathway or this disease mechanism. Is that kind of part of the idea? Yeah.
Alex Gurbych [00:23:04]:
Yes, that's exactly the idea. But from your permission, I would reverse the order. And AI is not at the end. AI is at the beginning. And it is connected with a range of assumptions and limitations. Assumptions. For example, you mentioned alpha fault. Now it's second with the latest improvement.
Alex Gurbych [00:23:27]:
But it is trained on the majority of crystal structures. There are open libraries with structures of biomolecules, invited small molecules, peptides and other things. The problem is that this structure usually is either infrared measured or Raman or NMR. And these methods, excluding NMR, require a matter to be a crystal.
Seth Earley [00:23:56]:
Right, right.
Alex Gurbych [00:23:57]:
So those are crystal structures. So alpha fold now knows how to build crystal structures. But did you, did you see crystal structures in our bodies?
Seth Earley [00:24:07]:
No.
Chris Featherstone [00:24:08]:
Right.
Seth Earley [00:24:08]:
That's that. Not that. That's not a naturally occurring structure of a bio molecule, right?
Alex Gurbych [00:24:16]:
So, yes, yes, this is what I'm talking about. So in the best case, this is educated guess of how it might look like, right? And I advise to start with this education, educated guess, because it is something. It is something. But believe me, I tried to synthesize what I predicted. I lost some amount of money. Cannot disclosure here, but I do not recommend going into trials right away. You generated something. First you have this educated girl.
Alex Gurbych [00:24:48]:
Yes. And then you validate it with like talking molecular mechanics, quantum mechanics, etcetera, etcetera. The last must be quantum mechanics, because of the computational power it requires, but it provides the most accurate results, possibly amongst everything, but it takes too much time. So at the end of this pipeline, you have the best gas possible at the moment and the best molecules possible in the moment. Now, about limitation in molecules, the second part. So I started from assumptions, right? And now limitation limitations is I can generate any structure I can imagine. It could be amazing, like amazing something amazing. Oligopeptide, the mRNA oligopeptide.
Alex Gurbych [00:25:36]:
It can give me a tremendous results on docking and validation and stuff.
Seth Earley [00:25:43]:
But then synthesize.
Alex Gurbych [00:25:45]:
Yes, yes. Who will synthesize it for me, right? It all ends up with chemicals providers, right? So I go to like in a min camp space, real space, or I go to wuji or go to somebody else and tell, hey guys, can you make this molecule?
Seth Earley [00:26:01]:
And they tell me, no.
Alex Gurbych [00:26:05]:
This is the case. This is the case because I did it once.
Seth Earley [00:26:08]:
In theory. In theory, this molecule would be perfect for your construct. In reality, there's no reasonable way to synthesize it unless you can find a bio, you know, a biosynthesis mechanism. But then you have to start doing gene editing and you know all sorts of things and, and you don't even know if it works, right? You don't even know if it works. Yeah. So, so you almost have to start with the molecular constraints and say, is this a synthesizable molecule as one of the constraints?
Alex Gurbych [00:26:41]:
Yes, this is exactly the fast I made. Now I start, okay, not, not let's generate a chemical structure, okay? Let's think what people can do. Then you train the generative models using the chemical spaces. Then using these generative models, you start generating stuff. But you know that somebody can do it, right?
Seth Earley [00:27:01]:
Right.
Alex Gurbych [00:27:02]:
Then you do predictive stuff, and then you do all the validations and only then you have some molecules.
Seth Earley [00:27:11]:
So are chemical providers also using generative AI to take libraries of existing compounds and predict whether they can synthesize these more complex structures, given protocols and given procedures? Do you know if that's happening in the chemical synthesis world? In the provider world, yes, it is happening.
Alex Gurbych [00:27:35]:
That's why we have a job. Luckily, good organic chemists, they are rarely good data scientists. They kind of sometimes try, they can build some pretty to simple to meet complexity models. But you know, what is mastership? Mastership is like, okay guys, you need this. And then it's something that, it could be very non trivial.
Seth Earley [00:28:03]:
I was in organic chemistry, organic synthesis lab was my worst practical lab situation. It is unbelievably hard to synthesize chemicals, even when you have a well known process for doing so. And when these guys are trying to come up with new novel ways of creating new compounds, my hats off to them, because it's alchemy as much as it is chemistry. It's art as much as.
Chris Featherstone [00:28:34]:
Alex, where are you getting the, you know, like are you fine tuning some of these models or are you just, you know, using some foundation models out of the box or these actual, you know, like who's, who's training these large language models to give you the, you know, the answers and the references and stuff back.
Alex Gurbych [00:28:52]:
We did everything. We fine tuned models, we used pre trains, we constructed our own, we published papers on that. Some of them, not, some were proprietary, I cannot talk about them, but we did every possible way.
Chris Featherstone [00:29:08]:
Are these open source models or what? I mean, is it.
Alex Gurbych [00:29:11]:
Yes, I have several open source, but they are not LLMs, they are graph, graph, graph models. A little bit better.
Seth Earley [00:29:22]:
What are the models that are most useful for life sciences and these particular areas?
Alex Gurbych [00:29:31]:
It depends. What would you like to do? If you want to screen through papers published on some topics and extract information, you go with LLMs, you stick them to Internet, you force them to download webpage, I don't know, Pubnet. You do information extraction? Yes. If you generate molecules, you work with the retrosynthesis. With any generative tasks, then you have to follow the molecular structure. The molecules is nothing but a graph.
Chris Featherstone [00:30:03]:
So keep going, keep going, because I've got a follow on to what you're saying. Go ahead.
Alex Gurbych [00:30:09]:
So when you work with molecules, and it is proven in many papers, the best class of ML models is graph neural networks. And since everybody now is LLMs on height, everybody tried to use LLMs everywhere.
Seth Earley [00:30:23]:
Let's use analyze here.
Alex Gurbych [00:30:25]:
Everybody likes them. Let's talk about Genii. But the graph neural networks still give better results in generative tasks, molecular property predictions and stuff like that.
Chris Featherstone [00:30:35]:
So that's what I was going to double click on, because you mentioned that before. Where you're using graph as one of your data techniques to actually figure out all the associations. And then I'm assuming a binary multi classification type of, of predictions in there, right, where the graph neural net where, because we've, we're seeing this also in the business world, right, for customer service type stuff and use cases as well as for like network, you know, network anomaly detection and predictability. But the graph environment is starting to become, in my mind, absolutely essential for a lot of these use cases, right.
Alex Gurbych [00:31:15]:
I mean, go ahead. In molecular world, everything is a graph.
Chris Featherstone [00:31:22]:
Yeah.
Alex Gurbych [00:31:22]:
And that's, you know, the phenomenon we're talking about is model. Model. By definition it is what is a simplified representation of physical reality, which you can like throw here and there, try this and that, and actually have a guess how reality works. This is a model, a simplification of reality. If your reality is a graph by nature, why using LLMs? Yeah. You need a graph.
Seth Earley [00:31:56]:
Yeah.
Chris Featherstone [00:31:56]:
Which is probably more cost effective as well. Right.
Alex Gurbych [00:31:59]:
But you need to know which graph and which phenomena you are working with. For example, let me give you a very simple example what molecules, there are overall molecular effects like polar surface, right? And there are local molecular effects like some group functionality like amino group or carbocidic group. And if your phenomenon is caused by local or global effects, you need different kinds of graph neural networks. For example, for global effects it is better, it's a graph info max. For local, it's attention networks, because you don't need all the nodes and edges, you need only particular ones. And you need to network to learn that and focus on that groups particularly. And you don't need much that much that the rest. So see where, here is where the mastership comes in, because you need to know all of these theme nuances.
Seth Earley [00:33:02]:
Yeah. One of the things, one of the things that you mentioned before is like searching PubMed and doing entity extraction or classification, that even requires not a large language model, but some kind of a language model that is usually used for classification and entity extraction. It can be an ontology or it can be a graph, a knowledge graph. But many times I've seen those models as too big and bulky. Like there was one that one of our pharma customers was using that was part of their search environment, their search pipeline processing. And that model had all sorts of life sciences related entities, but there were lots that they didn't care about, like animal diseases. They weren't working on animal diseases. Maybe there's an animal model of a disease, but not an animal disease, and that's just one example, but it had 13,000 terms across five facets.
Seth Earley [00:34:01]:
Right. That's kind of unwieldy. It's not possible to have good user experience with that. So even when you start looking at entity extraction classification, that type of clustering, you still need to, I think, fine tune that language model, which is again, different than a large language model because it doesn't have weights and biases and layers, but it's simply more of a set of controlled vocabularies that you're doing some type of entity extraction or classification. Again, you can do this from a semantic perspective, which is where a knowledge graph comes in, because you're going to relate. In fact, that's what it should be, because you can't use all those terms to just classify. You have to use conceptually related terms and again, bring it down to a, trim it down to a level that's more usable. So I don't know if you have any thoughts about those types of language models.
Seth Earley [00:34:59]:
Again, it's not the same as a large language model, but it is used for things like entity extraction classification, especially when you're looking at large amounts of literature.
Alex Gurbych [00:35:10]:
Thank you for this question. First, I have a question why they didn't start from putting together a vocabulary of terms that they needed and their relationships, because what you they should start from that they have to define the vocabulary, the relationships they are interested in, and then give it as a task to this large model, because this is how it works.
Seth Earley [00:35:32]:
I agree, I agree. I'm saying that the way that they did this was untenable, unusable, and part of the work that we did was to trim that down and to build it up from exactly what you're saying. But it's very common to see that you know, where especially search engines or search tools or various solutions have these pre built structures that are completely inappropriate to what they're trying to do. And I imagine the same thing is going to happen with large language models, where again, you have to either tune the content or you have to tune the model. And it's probably easier to tune the contents and to use something like a retrieval augmented generation.
Alex Gurbych [00:36:17]:
Okay, I got your question. So there are stages in LLM application and development, and there are sizes. This is another dimension. The larger the model, the more information it can capture. Right. But then the more useless information it will give you when you apply it. The smaller, the more also the larger the model, the harder to fine tune it. And for the largest ones, it sometimes you physically cannot do it because you need a hundred or 800 just so that it fits the model.
Alex Gurbych [00:36:51]:
And sometimes you need multiple of them. So if you have smaller model, then it can focus, it can capture less information, it can focus on some small narrow, the smaller the model, the smaller the topic with greater quality. But it will go off once you step aside. This topic. For example, if you extract information about amino acids, you can fine tune one model, so it does it, but then you can get also some information, which is wrong. But it is easier to fine tune.
Seth Earley [00:37:22]:
If you go beyond the use cases. So the model really should be constrained by the. Or the use case should be constrained by the model of the model. Constrained by the use cases, right. Because you're talking about amino acids and you're not talking about, you know, large biomolecules, right, or whatever it might be. Right. You know, but the point is, if you get off of the topic of that language model or outside of its training capability, then that's when you're going to get less accurate answers. So it's really aligning the use cases with the language model to get more precise.
Seth Earley [00:38:01]:
It's like you can't say the context is everything people talk about. Oh, just point your AI to everything. That's what people used to say. And I'd say that's like saying, you know, as a human, where do you go for answers? You go to specific places, for certain contexts. You go to a CRM system where you go to particular knowledge base or you go to a book in a library or you go to a particular expert, right? You're always looking for context and you're not, you can't just open up everything to everybody and then use an ambiguous query that's going to have all of these different possible interpretations. You're going to get more junk, right? Because you're broadening that context and you're broadening that scope and then asking ambiguous questions without enough fine tuning to get to the right place in that gigantic corpus, right? So that's why it's so difficult. That's why there are specialty websites for all sorts of things, right? You know, chemical abstracts and pubmed and you know, whatever and gene reference libraries and antibody libraries, right? And all of these things because you need that context. Because if you add a very, ask a very broad question across all these garbage, you can eat garbage.
Seth Earley [00:39:17]:
And that's why, and that's why one of the things that modular rag is trying to do is it's trying to make up for that lack of quality of content, right? By trying to get rid of redundant content and ambiguous content and irrelevant content. But it's kind of trying to make up for our sins in content curation and content hygiene and data hygiene.
Alex Gurbych [00:39:43]:
My comment is that I think, and you met this obstacle, is that in your case, people, okay, they heard about LLMs, they applied it somehow, then, oh, damn, how can we use it? And they called you and asked you to help. And you, as a surgeon, told them, guys, you started from the wrong side. You start from a use case vocabulary. Then you choose the tool, right? Like small, big, fine tune, rack, modular. It's all secondary. These are tools, right? You need to start thinking from the business case. Business case. Then you select tools.
Alex Gurbych [00:40:18]:
Or you can try to take a shovel and apply it everywhere, because everybody talks about shovels. Shovels are great nowadays. Yeah, let's apply them everywhere. Let's program with shows, right? No.
Chris Featherstone [00:40:31]:
Hey, Alex, let me ask you something. So, you know, given all the things that we've talked about, is there. Is there something that, you know, in terms of, you know, something you'd like to make sure that we all know, right? Something that's maybe nascent to you and what you're working on that we haven't covered before, we step into a little more personal questions about you.
Alex Gurbych [00:40:59]:
For example, I've been building several products. One for. I know that I have two tools in development. I know that China is separating now from us, and the majority of chemical synthesis is done. Like 30% to 40% is done in China. And now it will all move to Europe or us in this way more expensive. So we are developing a pipeline that optimizes chemical synthesis pathways. For example, you have a retrosynthesis tool, right? It can throw at you all the possible pathways.
Alex Gurbych [00:41:38]:
How to get this compound experienced chemists look at it and like, oh, garbage, garbage, garbage might be okay, I like this one. And then they select something or several, right? What they don't know is the cost of h, and they cannot. They kind of heal, but they don't know numbers of yield of a final product.
Seth Earley [00:41:58]:
Right.
Alex Gurbych [00:42:00]:
Retrosynthesis is not new, but if we apply to this cost calculation of synthesis and the yield throughput, like how much of a product you will get at the end, then it will be a way easier to evaluate pathways and it will give extra information. So this is one product that we developed, and another one is set, actually, on that conference, remember by it world, I heard from three or four companies, I hope this is not in the information. They wanted a particular structure for knowledge extraction, and we started to develop it in house because I think all of them need it. So this is the second internal proprietary product. Also, they have many databases and they are not connected. And we know a way how to interconnect them and work with this data in a smooth way.
Seth Earley [00:43:10]:
The other thing we had talked about before was quantum computing. And I know quantum computing is so still early stage. What are your thoughts about quantum computing?
Alex Gurbych [00:43:26]:
I think now it's not emerging technology. Now it's a research. It's not ready to do anything. In reality, only the simple model tasks. But now, as opposed to a few years ago, we have libraries, some standardized approach, we have available hardware to run some research tasks and stuff. And I think that in five to ten years, it will be another big thing. That's my opinion. Because drug discovery at the very end is you try to simulate the matter, and the matter is to the depth we know it are nuclei and electrons spinning around.
Alex Gurbych [00:44:15]:
If you model that, then you can model everything with an amazing precision. Schrodinger equation can be solved for three systems. It's a proton, hydrogen, electron and proton of helium. For other systems, it's approximated solution. When we have quantum computers, we can solve this system, this equation for every system, and we will know how each matter behaves. Exactly. This will change chemistry, biology, bioinformatics, chem informatics, all of that, material science in general, because we will be able to develop materials with a super high precision. Why? Because the tool works in the same way as nature.
Alex Gurbych [00:45:07]:
The position of electron in the system is undefined. The concept is atomic orbital. You know, what is it from the school? It's 90%. It's a probability. Exactly.
Seth Earley [00:45:21]:
It's a probability electron here. It has a higher likelihood here. So it creates this probability cloud of these different shapes would be these orbitals.
Alex Gurbych [00:45:30]:
Yeah, but kind of, we don't got.
Seth Earley [00:45:32]:
A deal at every level, and then they change in combination with other molecular structures, and then that becomes very, very complex. And the combinatorial explosion of those probabilities is just infinitely more, requires infinitely more computing capacity than we could possibly have on the planet.
Alex Gurbych [00:45:55]:
Exactly. Exactly. In the. In this system, you move one electron, it affects all the system, and you have to recalculate, recalculate all the system particles. And having recalculated every other system particle.
Seth Earley [00:46:10]:
You have to recalculate all the others.
Alex Gurbych [00:46:12]:
And it never ends.
Seth Earley [00:46:14]:
Right.
Alex Gurbych [00:46:14]:
See, that's the mad. It's a madness.
Seth Earley [00:46:17]:
Yeah.
Alex Gurbych [00:46:18]:
How quantum is different for them, because quantum works exactly in the same way. It's also particles with some probability, and you can emulate that efficiently. Yeah, the same story.
Seth Earley [00:46:36]:
Seems very elegant. It seems very elegant in concept, perfectly. Yeah, that's so. I've never thought of it that way. I've never thought of it that way. I've always thought. Yeah, go ahead. Keep going.
Alex Gurbych [00:46:48]:
So it's same with graphs and molecules. The graphs are the perfect model of a molecule, and quantum computer is a perfect model of matter over nature.
Seth Earley [00:47:00]:
Wow. That's going to be quite. Quite something down the road. And there's really a lot of great stuff to look forward to. You know, just a little bit about your background. I know you're quite an athlete. What do you do for fun these days? Are you still. Are you still.
Seth Earley [00:47:17]:
You did some competition in the past in terms of powerlifting. Talk a little bit about what you've done for fun and a little bit more while you're getting your two PhDs. And is it three master's degrees or four master's degrees?
Alex Gurbych [00:47:32]:
Three. Three.
Seth Earley [00:47:33]:
Sorry, I didn't mean to oversell you there. We're also doing some other stuff. Tell us about that.
Alex Gurbych [00:47:41]:
My wife. My wife says that I could do something, you know, useful for the. For the. For people or for work, instead of sitting in front of books for all the years.
Chris Featherstone [00:47:51]:
Yeah, that's funny.
Alex Gurbych [00:47:55]:
No, no, that's. That's a joke. That's a joke, of course, because I hope that it helps me to understand, to combine, you know, cross disciplinary knowledge, at least in drug discovery. It's not enough to understand one side. It's not enough to understand biology and not math. It's not understand. To understand math and understand chemistry, you know the story. You have to understand it in a complex and it can give you some direction where to move.
Alex Gurbych [00:48:30]:
Speaking about my hobbies or sports. So I'm master of sports international class in powerlifting. I finished competing about three years ago when I got kids. At that time, I did everything I wanted. I became absolute champion of Ukraine. And, like, master of sports international class, I got all these labels, chevrons, if you want. And I stopped competing. I stopped competing because it's destructive for health.
Alex Gurbych [00:49:03]:
You know, you have blood in your eyes because the weights are too large and it's. Yeah. And then I did what I were.
Seth Earley [00:49:11]:
What were your competitive presses or exercises? What were you most. What were you most well known for?
Alex Gurbych [00:49:22]:
My last competition, I was 90 kilo bodyweight. I squat 300, I benched 200, and I pulled 300 in one.
Chris Featherstone [00:49:33]:
Kilos, right? Kilos, yes, kilos.
Alex Gurbych [00:49:36]:
Not.
Chris Featherstone [00:49:37]:
Yeah, that's. Yeah, that's two. Yeah, 2.2. For those listening it's 2.2 pounds per kilo.
Seth Earley [00:49:42]:
So you, so you squatted 600. 600 pounds.
Alex Gurbych [00:49:45]:
Yeah, almost 600.
Seth Earley [00:49:50]:
660 would it be? I think 660 pounds. And then you, you benched 440.
Chris Featherstone [00:50:00]:
Yeah, just a little bit of weight, right? Yeah.
Seth Earley [00:50:02]:
For 90 kilos. Yeah.
Chris Featherstone [00:50:05]:
In Alex, in the US, you'd be known as, as part of the 1500 club. Right.
Alex Gurbych [00:50:09]:
So 660.
Chris Featherstone [00:50:11]:
Yeah. So squat plus bench plus maybe a clean or something like that. Right. Over 1500 pounds. So, yeah, combined it's combined, yeah. So it's impressive. Very impressive. Especially at 90 kilos body weight.
Chris Featherstone [00:50:26]:
Right. Which is even, you know, more impressive because the, they believe that the Olympic lifters and the, and the power lifters are the strongest people on the planet if you can lift twice your body weight. Right. In various lifts. So that's impressive.
Alex Gurbych [00:50:40]:
Yeah, it's nice.
Chris Featherstone [00:50:42]:
You didn't stunt growth. Right. And now you're, you know, used to be six foot, now you're five. Five.
Alex Gurbych [00:50:49]:
I will never know how tall, how tall could I grow? But I cannot do that anymore. I cannot anymore.
Seth Earley [00:50:58]:
Part of your body? Part in your body.
Alex Gurbych [00:51:01]:
Yeah.
Chris Featherstone [00:51:01]:
It's impressive, though. It's very impressive.
Seth Earley [00:51:03]:
Yeah, that's great. Anybody you do now for fun, do you? Mostly occupied by the kids. How many kids do you have kids?
Alex Gurbych [00:51:12]:
Day three and five. And this is the only thing that I do in my spare time.
Chris Featherstone [00:51:18]:
Yeah.
Seth Earley [00:51:19]:
And wonder why you have no spare time. Well, this has been wonderful. Really, really pleased that we met at the bio it conference. You had asked wonderful questions in our workshop and I knew I had to have you on a podcast, and this has been a lot of fun and we're certainly kindred spirits. I've never competed in olympic level or powerlifting championships, but I certainly try to keep myself in shape. So my hat's off to you for those kinds of accomplishments. That's really fantastic. Well, listen, Alex, thank you so much for being here and sharing your expertise and spending some time with us.
Alex Gurbych [00:52:02]:
Thank you, Seth. I appreciate your questions and your invitation, and it was also a great pleasure for me to meet you and talk to you. And let's look, how can we collaborate? Absolutely.
Chris Featherstone [00:52:16]:
Much success to you in the future, my friend. There's so many wonderful opportunities. I hope you get your lion's share of it.
Seth Earley [00:52:24]:
Yeah. And we'll look forward to working together in the future. And again, thanks, everyone, for tuning in and for listening. This has been another episode of the early AI podcast. Today we covered some really wonderful topics and we'll continue next time while we're exploring the transformative effect of AI across industries. So thank you, and we will see you next time.
Chris Featherstone [00:52:48]:
Thanks, Alex.
Alex Gurbych [00:52:49]:
Thank you, everybody. Have a good day.
Chris Featherstone [00:52:51]:
Thanks, Carolyn. Have a great day. See you guys.