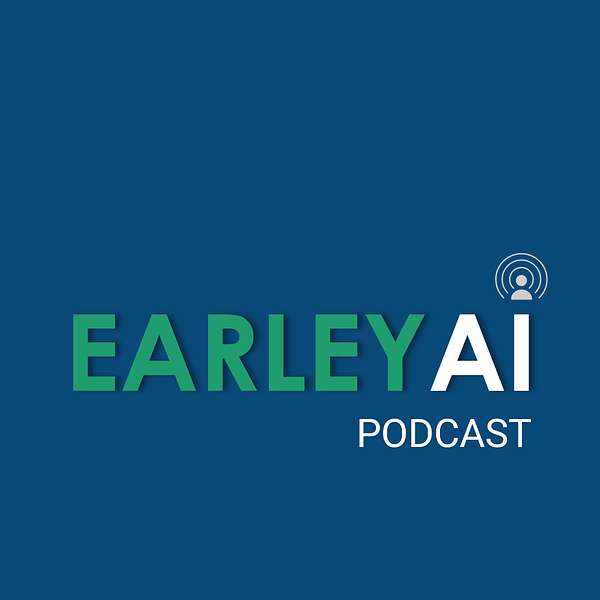
Earley AI Podcast
In this podcast hosts Seth Earley invites a broad array of thought leaders and practitioners to talk about what's possible in artificial intelligence as well as what is practical in the space as we move toward a world where AI is embedded in all aspects of our personal and professional lives. They explore what's emerging in technology, data science, and enterprise applications for artificial intelligence and machine learning and how to get from early-stage AI projects to fully mature applications. Seth is founder & CEO of Earley Information Science and the award-winning author of "The AI Powered Enterprise."
Earley AI Podcast
AI, Knowledge Management, and Navigating the Hype with Daniel Cohen-Dumani - The Earley AI Podcast with Seth Earley - Episode #050
With extensive experience in AI and machine learning dating back to 1998, Cohen-Dumani brings valuable insights into the historical and present-day landscape of AI, emphasizing the importance of foundational knowledge, expertise, and knowledge management in making AI work effectively within organizations.
Tune in to this enlightening conversation as they discuss the attention and resources that must be invested in unstructured data and knowledge to leverage the full potential of AI.
Key takeaways:
- A foundational reference architecture is critical for making sense of data and discerning between vendors' aspirational capabilities and reality.
- Traditional long-term technology planning is no longer applicable in the age of AI and large language models (LLMs) due to the unpredictable nature of AI's uses and leveraging capabilities.
- Executives should personally experiment with AI tools and allow more freedom for workers to adopt AI, rather than stifling innovation.
- Building an extensible and expandable data foundation and good enterprise architecture is crucial to avoid data silos and maintain consistency in data.
Quote from the show:
"I think one of the challenges that organizations have is they're not investing the time, the effort, the money, the resources, and the attention on unstructured data, on knowledge. You know, if you look at any accounting department, they spend inordinate amount of time and resources on numbers, on transactional data. But if you look at how much effort is put on unstructured data, it's night and day. And yet unstructured data is 80+% of the data most organizations have." - Daniel Cohen-Dumani
Links:
LinkedIn: https://www.linkedin.com/in/dcohendumani/
Website: https://www.withum.com
Thanks to our sponsors:
Welcome to the early AI podcast. My name is Seth early. Unfortunately, Chris Featherstone couldn't make it today. I'm really excited to introduce our guest. For today, we are going to be discussing the ways of interacting with computers and technologies and conversational ways. We'll talk about the need for high quality data, talk about data architecture, knowledge architecture, and how AI is going to be impacting organizations short term and long term.
Daniel Cohen-Dumani [00:00:37]:
And we'll talk about how we need to think about increasing the AI literacy within the organization and driving the urgency of adoption to take advantage of these AI opportunities. So our guest today comes with a huge amount of experience. His company was acquired I by Witham in the last seven years. He's originally from Switzerland. He's now a longtime resident of the DC area, and he's at across roads where he's transitioning out of his current role and contemplating what he might do next, which may involve doing some work in the SAS space. He has deep ties to the technology sector. He has a history of expertise and knowledge management. Daniel Cohen Damani, please welcome.
Daniel Cohen-Dumani [00:01:25]:
Welcome to the show. Thank you for joining us.
Seth Earley [00:01:28]:
Thank you, Seth. It's a pleasure to be here. I know you and I have known each other for quite a while and I'm excited to be on your show.
Daniel Cohen-Dumani [00:01:35]:
Yeah. How long has it been, would you say?
Seth Earley [00:01:37]:
I want to say 1015 years for sure.
Daniel Cohen-Dumani [00:01:39]:
Yeah, yeah, that's right.
Seth Earley [00:01:41]:
In many occasions over the last 20 years.
Daniel Cohen-Dumani [00:01:44]:
Yeah, yeah. It's really been nice to reconnect with you. So I want to start off with some of the misconceptions around one of the spaces that you have a lot of expertise in experience with, which is knowledge management. And what would you say? What are the misconceptions today versus five years ago, ten years ago? Or are they the same or how are they different? What's going on in the space these days? What do people not get?
Seth Earley [00:02:11]:
Yeah. So it's interesting because I've seen the world. I mean, knowledge management has been around for almost 30 years, but you and I know that it's been, it's been a goal of organization to be able to better manage their knowledge for a long time. And technology have come along the way over the last 25 years and have mean incremental improvement. But some of the misconceptions that I've seen is thinking about knowledge management as an end into itself. Right. It's not, you know, there's a buzzword, right? And we used to talk about knowledge management, then people say, hey, let's not talk about it anymore. Now it's coming back.
Seth Earley [00:02:52]:
It has a negative connotation. As you know, the km thing, it's not an end into itself. It's an intent to leverage and build market value for your organization and give you competitive strength. That's what knowledge management is all about. There's often a myth about, hey, we're going to just hire smart people and that's going to solve everything, right? Are we going to put process in place and that's going to solve everything. There's also a myth of, hey, let's buy very expensive technology and that's going to solve everything. And those have been around for a while and it's really haven't changed. What really have changed is the advance in AI.
Seth Earley [00:03:39]:
I think AI is changing everything for knowledge management, but obviously for everything else. Right. So that's something that really struck me.
Daniel Cohen-Dumani [00:03:48]:
Yeah. And I totally agree. You know, if you're too far removed from the customer, if you either have to serve the customer or serve someone who's serving the customer with your knowledge initiatives, otherwise it's too academic, right. It's too theoretical and you get a lot of navel gazing, a lot of definition, let's define knowledge management. But you did make an interesting comment. You said sometimes people say, well, let's just hire smart people or let's just put a process in place, let's just buy technology. That is the people process technology. So it has to be some combination of those, right? So what is missing when you say that? What is missing is it that they try those things independently, but it's really a holistic approach or what else is missing there?
Seth Earley [00:04:30]:
You know, I think, I mean, part of it is having a clear strategy of why you want to do this, right? And thinking about, okay, knowledge management is great, but what are the benefits? What are the outcome? Why are we doing it? It's the why, why the typical why you're doing this.
Daniel Cohen-Dumani [00:04:48]:
I like to say the so what when you have so what. Yes, exactly.
Seth Earley [00:04:53]:
So what. And not just doing it because other people are doing it. Right. So I think, yes, it is a people process technology, but it has to be combined. It's all of it together. Very important aspect of change management with any technology and specifically around knowledge management.
Daniel Cohen-Dumani [00:05:12]:
Right, right. And so when you talk about how AI is changing things, now, when I first saw chat GPT at the end of last year or mid last year, or whatever it was, I had a little bit of an existential crisis. I was like, oh my God. Is my work in knowledge management and information architecture no longer necessary? And of course, the more research I did, the more I realized that it was more necessary than ever. And so start looking at that, like, what do you see in that space? And then I still look at the fact that all the things we're trying to do with technology is to make up for our past sins in poor data content and knowledge hygiene. So when you say AI is changing things, in what way? And what is the role of knowledge management at this point?
Seth Earley [00:06:04]:
Yeah, that's a great question, Seth. And if we take a step back, when you say chat GP, that's large language model. I like to make sure everyone understand what is a large language model? It's a process in technology and algorithm that being trained on all publicly available knowledge in the web. Publicly available is important so we can think about it as general intelligence. It's generally available concept, right? You can ask a question, you get an answer, and it's usually pretty accurate, although it elucidates quite a bit. But it's usually, hey, it sounds really plausible, right? It sounds really good and it sounds like it should be true. Right? When we think about knowledge management and how LLM is impacting it, now we have a technology that can be pointed to your content, specifically your document, and one by one, understand what's in it or start extracting important content. That's really what the game changing is.
Seth Earley [00:07:12]:
So the game changer is now we have a very extremely unique, powerful technology that can read unstructured content and start making sense. I won't say reasoning, we're not there yet, but at least start making sense and extracting concept and entities, and relationship of entities, and start doing manipulating content of unstructured documents.
Daniel Cohen-Dumani [00:07:39]:
Yeah, and so it's interesting. So what you're referring to is generically called rag or retrieval augmented generation, right? Because of ground truth in the enterprise. And what I have seen and heard and read and researched is that that use of LLMs is going to have a greater impact on the enterprise than any other AI initiative. And I agree with that. I think that's why executives are not necessarily thinking of large language models in that class of technology correctly if they are just using it to answer questions without reference to corporate knowledge. Because again, it can only do so much. It doesn't know your products, it doesn't know your services, it doesn't know your solutions, it doesn't know your competitive advantage, it doesn't know your differentiating ip and you don't want to let that out. You want to keep that safe.
Daniel Cohen-Dumani [00:08:38]:
And so doing this in such a way where you can use it to understand what the user is asking for, facilitate a retrieval, and then make that answer more conversational, that's brilliant. That reduces so much work. But it's still thinking of the large language model capability differently than what a lot of organizations are doing. A lot of organizations are not necessarily thinking of it that way. They're trying to get get it. I mean, I think it's coming along, right. I believe that people are realizing that, but I still think that transition to that thinking is a little bit slow. And then tell me more about the role of knowledge hygiene, knowledge process, knowledge architecture in that situation, because it still does obviate the need for that stuff, right?
Seth Earley [00:09:29]:
It does not, absolutely not. And I think it makes it even more important. Right? So the first thing you can think of is content security, right? As you know, there's been number of stories of organizational enterprises starting to use LLM against their data. And what they realize is people have access to more things they should.
Daniel Cohen-Dumani [00:09:52]:
Ah, yes, right.
Seth Earley [00:09:53]:
So that's content hygiene or content governance and security. Well, guess what? They had access to it before, they just couldn't find it. So the LLM made it easier to find it. But they had access to this magic spreadsheet with a lot of salary data that they were not supposed to. They just couldn't find it. They didn't know it was there. Nowadays, because the ALM are really good at understanding what you want and trying to find the best results based on your question. But when you think about data, more structured data, which hold a lot of information that LLM can make sense of as well, bringing back this concept of data architecture, and it's not just in structure, it's structured data.
Seth Earley [00:10:39]:
It's thinking of data structure and structure as one big data architecture and thinking about where is the data, what's relevant. If you're in a business of serving customer, where's my customer data? In how many place do I have it? What's the master record for that customer data? And the LLM needs to know that, otherwise they're going to find a lot of things and they won't know how to prioritize those. It's bringing that need to clean up, organize your data. More than ever, we're seeing a lot of demand from organization to create a comprehensive data architecture as a result of AI advances.
Daniel Cohen-Dumani [00:11:25]:
So they are making the connection to that. They are putting the funds in there. They're starting to realize the need.
Seth Earley [00:11:31]:
Yes.
Daniel Cohen-Dumani [00:11:32]:
Yeah, yeah. And of course, it can be very significant. So that says that there's some organizations that are doing that. What about, what's that level of awareness generally look like? So that sounds like that's a forward looking company that understands the need for the data architecture. They understand the need to do some content processing or content curation, but that seems like it's not a big part of the space. What percentage of executives or companies would you say have that level of understanding, awareness and commitment to the foundation versus the ones that are kind of what is the.
Seth Earley [00:12:14]:
In my personal experience, speaking with a lot of executive over the past 18 months, 24 months, I'm still floored of the lack of awareness and priority of data and AI in those businesses. I want to refer back. And then when you think about it, GPT four was released a year, a year and a half, like 14 months, 16 months away. It's only been that long, right? You know, Bill Gates wrote an article in November of 2023, which is seven, eight months ago. We're in June now, 2024. And the title of this article was AI is about to completely change how you use computer. And we can like or dislike Bill Gates. I think his prediction tend to be fine on target.
Seth Earley [00:13:01]:
And he said, and I quote, in the next five years, this will change completely. You won't have to use different apps for different tasks. You'll simply tell your device in everyday language what you want to do and your device will do it. And depending on how much information you choose to share with it, the software will be able to respond personally because it will have a rich understanding of your life.
Daniel Cohen-Dumani [00:13:26]:
Right.
Seth Earley [00:13:27]:
In the near future, anyone who's online will be able to have a personal assistant powered by AI that's far beyond today's technology. That's a five year prediction, actually, four and a half year prediction, when you think about that, is the word of agent. Agent is something we hear. AI. Agent and agent is just a software that responds to natural language that can accomplish many different tasks. So for those executives, they don't understand that this world of being in front of a computer, typing something, emailing someone, getting on a Zoom meeting or teams meeting or getting on slack or teams messages, that's going to change, right? Dramatically. It's already changing now. And I think there's a broad lack of understanding of this impact.
Seth Earley [00:14:19]:
And when you think about it, this is impacting every single business. You're going to be able to talk to a machine that will know who you are, know what you want, and you'll be able to tell it to accomplish discrete task tasks that clearly you ask someone else to do.
Daniel Cohen-Dumani [00:14:38]:
Right.
Seth Earley [00:14:39]:
You'll delegate your work to agents as opposed to delegating to your co workers. Right. So, you know, for me it's, you know, executive are still in their bubble, right? Oh, I get chat GPT or it came out. And I think they're still thinking the same way they've done it in the past 20 years. New technology. Let's do a pilot, let's give it to it, and it will figure out how to roll it out. Let's block everyone to use anything else because we're worried. I think AI is fundamentally different.
Seth Earley [00:15:14]:
There's a lot of study that has been made that AI that's well adopted comes from a grassroots approach. People start using it with no constraint and trying to find ways to use AI to their benefit, as opposed to a CEO coming and said, this is how you're using AI, which is sort of this big shift in approach to technology that I see a tremendous lag.
Daniel Cohen-Dumani [00:15:43]:
Yeah, yeah. And I want to make a quick comment about your anecdote or your commentary about security. We were doing a project for a pharmaceutical company, and we built a portfolio review application, did all the metadata and the information architecture, and this application reduced the need to go to, like five or six other applications and multiple spreadsheets. It did all that stuff. All the motherhood in Apple pie centralized this and made it easier to consume, and we componentized the content, et cetera, et cetera. So great application. Highly, highly, highly sensitive information market moving information, very tight security on this. Executives, not even every executive on the leadership teams were able to see some of these things, right? So there are some things that were so sensitive about acquiring companies or, you know, clinical trial results that even at that level of senior leadership, not everybody could see it.
Daniel Cohen-Dumani [00:16:42]:
So very, very locked down. The company installed Copilot, Microsoft Copilot. This is built in a sharepoint application. And then we got a frantic call on like a Friday night or a Saturday and saying that, you know, that this new copilot agent is able to access all of this information in this application, and they're freaking out. Of course, this became our urge, you know, our emergency to fix it, but we had to. We had to lock it down even further and remove. But the point is, nobody anticipated that, right? They're saying this thing's going to have admin rights across all these applications. They didn't think about the fact that it shouldn't have admin rights across all those.
Daniel Cohen-Dumani [00:17:27]:
And that this is sensitive information that shouldn't get out. Now, luckily, we were able to capture captured. I had to go drive over to my developers home on Saturday to find him, but we got it fixed. But I think it's that point of saying, you know, yes, we have to be careful about this because we can't just give them access to everything because then we're giving everybody access to everything. So I just want to make that a quick commentary. And then, you know, you were talking about how they need to think about the fact that this is going to be completely changing how we interact with computers and technology, and we're going to be asking questions of agents, delegating tasks to agents and so on. But from getting, getting from here to there is going to be a bit of a hike, right? There's going to be a bit of a trip. So how should organizations think about their investment in the space today? Because there's a lot of stuff that's foundational that needs to be put in place.
Daniel Cohen-Dumani [00:18:25]:
So where should they be to go along on that path? What should they be doing? Now.
Seth Earley [00:18:33]:
A very smart person said, your job is not at risk, but your job will be lost to someone using AI. And I think the same has to be true for companies. The company that will survive, and we're talking survival here, are the ones that are able to embrace and leverage AI to their competitive advantage. So this is. We're talking about survival, but it's not just an existential.
Daniel Cohen-Dumani [00:19:05]:
It's existential.
Seth Earley [00:19:06]:
Existential is the word, yes. You know, I feel like, you know, if you're an executive and you have not, you have not experimented yourself if you're not using Hrgpt or copilot. I'm a big fan of perplexity. I use perplexity on a daily basis. This is my go to AI. I mastered it. I use it. But if you're an executive and you're not experimenting yourself, you are never going to understand the impact, right? Because at the end, you need to really understand it yourself that you have your own copilot next to you that can do tasks that were really hard for you, including explaining to you those concepts that you don't understand.
Seth Earley [00:19:57]:
Go to copilot today if you're an executive and ask, what are the threat to my business? My business is x, Y and Zenith. If you don't embrace AI, and guess what? You'll get a very accurate, good answer. Maybe a little hallucinating, but no. For the most part, it's going to be a good answer. So I think my advice to executive is experiment yourself. Otherwise you're doing your company and your team a disfavor. Second, I would say, don't try to stiffer innovation or block innovation by saying we can only do it this way, right? Because guess what? People are going to find ways to do it on their own using whatever tool they want. That's just the nature of people, right? And there was a study from Microsoft recently that said that 70% of worker have been using AI, which is staggering when you think about it, right? And most of them are doing without anyone knowing they're doing it.
Seth Earley [00:20:56]:
Which means they are likely putting a proprietary data into publicly available LLM and doing things they're not supposed to. But guess what? They're trying to do their job better. So I think giving more tool and more freedom to worker is how you're gonna become, you know, AI ready or AI first company. Otherwise you're gonna be, you know, trailing, trialing behind.
Daniel Cohen-Dumani [00:21:26]:
Yeah. You know, I think one of the things that, that is very challenging is that, you know, there's less of an understanding of the need for a good, solid data foundation, right? I mean, it's there. People are giving it lip service as they always have, right? You know, sit around the board table, they're like, oh, yeah, data is important. Everybody nods their head, yeah, data is super important. Yeah, we live on data, right? And then you put in front of them the investment that's required. They go, whoa, it's not that important. So there's this disconnect between this kind of conceptual understanding that data is important and this visceral understanding that you have to spend money to fix it. Then, of course, people have been burned, right? Executives have been burned before.
Daniel Cohen-Dumani [00:22:11]:
So how should they tend not to invest in these data foundations? And when you do, it's usually focused on an immediate project, which makes sense. But how should they think about data foundation across projects? Because again, that's where we end up with more silos, more inconsistent organizing principles, more bespoke architectures, and again, it further fragments knowledge and data and content in many cases, unless we think of kind of a common layer. So what are your thoughts about that? You don't want to blow up the scope too much, right? You have to keep the scope focused. But what are your thoughts about building that in a way that's extensible, expandable, that enables cross application communication and data flows and so on? What are your thoughts about that?
Seth Earley [00:23:10]:
Yeah, it's a great question. Seth and I've experienced a lot of different scenarios that are similar to this. And I always say it starts with a good enterprise architecture. And what I say that is the reason there's silos and systems not connected is because there's urgency to fix a problem. And we say, okay, we're going to buy this software, it's going to solve my problem. It's going to be great. But we're not thinking about the impact.
Daniel Cohen-Dumani [00:23:39]:
Of the rest of the organization downstream, upstream processes, data.
Seth Earley [00:23:45]:
And that's of the challenge of software as a service. It's so easy to put a credit card by the software and solve the problem, and all of a sudden you have data here that exist here and they're not connected, and that creates a problem. So I think enterprise architecture of understanding why you're doing this and how you're doing it is the impact on the rest of organization is something that small, medium, even large organization don't have a good handle on. Right. Because there's a need of speed. Right. There's a need of, I need to solve this problem now. Right.
Seth Earley [00:24:19]:
And I think, you know, when you think back over the last ten years, be damned.
Daniel Cohen-Dumani [00:24:24]:
Anyway. Sorry.
Seth Earley [00:24:25]:
Yeah, yeah, yeah. Technology debt, and I think we've gone through some economic cycle. Of course, the pandemic upheld everything. So there's been many companies that have put off saying, you know what, this is not the most important thing. I'm going to wait and wait and wait. And now with AI coming in, they say we can't wait anymore. So it gets back to organize and think about your enterprise architecture so that you have a data architecture, so that you can have a good foundation. Now there is a light at the end of the tunnel, and there may be some hope that advances in artificial intelligence will be good enough to say, you know what? You can have all those systems.
Seth Earley [00:25:15]:
I'll be able to make sense of it. I'm just going to take all the data and disambiguate everything and I'm going to fix it all. You and I've been talking a lot about knowledge graphs and the ability to leverage knowledge graph to do this. So there may be a hope down the road where it will be okay to continue to have this silos, but will be able to bring it back together because of advances in AI. But that should not negate the need to do it. It's important for any organization that have transactional system, they need to have consistency in data. Otherwise your transaction may not be accurate or what you do may not be as optimal as possible.
Daniel Cohen-Dumani [00:26:00]:
Right? Yeah. And it's interesting that more effort and attention and resources have gone into that transactional side, because again, so much is dependent upon that and people get measured on that and financial results need to be accurate and timely and all of that stuff. But imagine, but then when you start looking at data, a content and unstructured information and knowledge, you know, you don't have people. Imagine if people say, oh, I don't wanna tag this, or I don't wanna do that. Imagine if you had people in the accounting department going, oh my goodness, all those numbers, you have to put them in the systems, they have to add up. Right? You know, I mean, you don't think of the same way because it's just how the business is run and there's people whose jobs it is to do that. And we've so lacked that in the unstructured side. But to your point of will we get to the point where we can ingest all that data and then let the AI make sense of it? I think that to a degree, yes, but with the right reference architecture, I still think you need to have that foundational on top of it.
Daniel Cohen-Dumani [00:27:07]:
Foundational is critical, and then you may be able to do that. We showed that example with product cleansing and enrichment the other day where we're ingesting all of these different data sources into a vector space, but we're doing it along with the knowledge graph and the, and the ontologies for that particular organization. And then with that you can bring in these diverse sources and then you can prompt the LLM to using a templated prompting. Right. Using a template with variables to say, give me this information about this product, and then run that iteratively and produce cleansed data. So it is possible to do that, but under certain narrow circumstances. I think to your point about the enterprise architectures, we have to start with that domain model which says, here are the big picture organizing principles. This is what everybody needs.
Daniel Cohen-Dumani [00:28:04]:
And then you start digging down more deeply into these specific application areas, but recognizing that all of this stuff has to be harmonized. So, yeah, totally agree that it's an area that is moving so quickly, it's almost hard to say where you should place your Bethenne, you know, do you have thoughts about, about that? You know, because there's a lot of things that, you know, vendors will promise or say they can do and, or have a capability. I like to call those aspirational capabilities. Right. Yes, planning on it. They may speak about it in the present tense, but it's in the future. It needs to be in the future tense. They don't have it today.
Daniel Cohen-Dumani [00:28:44]:
Right. I would say they're outright lying, but I'm going to give them a little benefit of the doubt and say they're aspirational capabilities. Like they're almost there, but they're not there. So how do you tell the difference between the vendor B's, also known as aspirational capabilities and reality? So what's the due diligence? Or what's the approach that organizations need to take to kind of separate what's possible from what's practical and what's real?
Seth Earley [00:29:11]:
That's right. That's right. Yeah, you bring a good point, Seth, and it's interesting because we are in a VAR different age and everyone call it the age of AI. And I think the mental approaches we had in the past specifically for technology don't apply. We used to be able to say, let's make prediction over the last five years. Let's create a five years technology plan. You can't do that anymore. Frankly, neither you and I have any idea where are we going to be a year from now.
Seth Earley [00:29:47]:
We can make prediction guess. I think Bill Gates made a long term prediction. It may not be five years, maybe seven or eight or nine, but it will happen. When you think about AI in a specifically large language model, it's one of the first technology that is being used for things that were not intended to. Usually you build a technology, you use it because it's going to do this. Now even you ask OpenAI and Sam Altman, he said, I'm amazed how people are using cha. We never thought it would be used that way. That's a very important thing to remember.
Seth Earley [00:30:31]:
We are in an age now where technology is coming. We literally have no idea how we can use and leverage it. Back to your question on vendor hype. Yes, there's tremendous amount of hype. Yes. When you think about all the vendors that are talking about, hey, we're doing retrieval augmentation generation rag on your knowledge and it's going to solve everything. That is not true because rag is still suffering from the same challenge that LLM had a. It's grounded on your data, but it's still going to make things up or importantly, not find what you want.
Seth Earley [00:31:10]:
Knowing that it's there, you know it's there and I've done it. I'm sure you experimented with copilot and I'm using copilot and I'm asking a question and I know it's there and it's not able to find it. Right, because my prompt wasn't long enough. Right. Or I wasn't specific enough or just it couldn't connect the dots. Right. Because we're talking about a lot of time. You ask questions, you ask about connecting the dots.
Seth Earley [00:31:34]:
LLM are not great at connecting the dots, too. So I think that, you know, people have to be careful when they hear we're AI, we're using LLM and we're doing things that looks very intelligent. I will be very wary of that because that's likely, you know. Yes, it will become very intelligent. Not there quite yet. I think we're going to hit a plateau of the capability of LLM. Right. When we think about LLM, they've been trained on all the content available, but there's not enough new content to train those large language model on.
Seth Earley [00:32:10]:
Yeah, you can do it now and you'll incrementally get more content, but that's it. The content has been ingested. Right. We've got everything, right. And the transformer architecture has been optimize and optimize and optimize. So how much more can we do? There's going to have to be something else coming because at some point it's going to be very challenging to optimize the current architecture. And we know some people are working on new ways of improving or getting better answers that LLM can do today.
Daniel Cohen-Dumani [00:32:50]:
Yeah. It is interesting when you think about all of the content and data that has been ingested, and there have been articles and podcasts about the fact that we're running, that the big AI vendors and platform providers are running out of data to ingest. And so where else can that data come from? And again, this is where they're not ingesting your proprietary content, right. Because that's your proprietary content. And so that's where know, ingesting that and accessing that is where, where the advantage comes from. But there's also, you know, because so much content is being created by these LLMs flooding the Internet. There's also what people have been talking about in terms of model collapse. In other words, the data is not diverse enough.
Daniel Cohen-Dumani [00:33:40]:
It's repeating. It's like the dragon eating its tail. Right? It's just, it's ingesting its own output. And that's, and you're not injecting creativity into the process. So, you know, again, whether that's, whether and when that's gonna happen, I mean, a lot of times you can just tell when the content is AI written, right? Because it has that certain cadence to it. And there are tools that will identify whether it's AI generated content.
Seth Earley [00:34:09]:
You bring a great point. It's one of those unintended use of llmdeh is the generation of what we call synthetic data that have been created. But it's very useful in a data strategy where you don't have enough data and you need your data set. You can use LLM to create data for you. So you can train machine learning algorithm on data that you don't have. Right. So, for instance, if you have proprietary data that you want to train, a machine learning algorithm is you can use LLM to anonymize that data set so that your proprietary information or client name or Phi Pih, whatever, HIPAA compliant data set is anonymized and removed. And LLM are great at doing that.
Seth Earley [00:35:02]:
Right. So that's one of those, you know, unexpected use of LLM. But yes, you know, the gulf of data when, when those large language models can be trained on synthetic AI generated content. We don't know what's going to happen yet.
Daniel Cohen-Dumani [00:35:19]:
Yeah, yeah, no, that's a really good point. So when you think about literacy and AI literacy and how, I mean, again, everybody, there's someone said to me the other day, there's like, everyone's an AI expert now, right? Like there's all these, you know, consultancies and people and so on. And, you know, I like to point out that I wrote a, co authored a book on IBM and Lotus's first foray into AI and machine learning back in 1998. It was on something called Lotus Discovery Server, which then became IBM omnifind, and then that DNA went into Watson and again had been writing about this stuff recently. We used to call it text analytics, remember? You know, there's. Now it's AI, but it was still the same principles of doing that. And they used to say that in the two thousands, there was a phrase, no AI ever works, because as soon as it works, we call it something else. So AI by definition doesn't work.
Daniel Cohen-Dumani [00:36:25]:
That was the saying back in the two thousands. But because word processing was AI, search always used AI, spell check was AI, speech recognition was AI. All of these things, we're using those algorithms. But word processing was one of the first applications. We don't say, I'm going to use my AI to write this document, it just became word. But it took human expertise and put it into a program, into an algorithm. So that was considered AI at the time, but it's been around for a long time. But they think the bigger pieces that are more critical to this are the knowledge pieces, the knowledge in data hygiene and the socialization, and the fact that we've been operationalizing knowledge management for companies for 25, 30 years.
Daniel Cohen-Dumani [00:37:14]:
And that's the level of expertise that I think people don't realize that you need in order to make these things work, because it does come back to that foundational stuff. Yes, you can layer on these different technologies in different ways. There are some nuances to it, but it comes back to the basic blocking and tackling of process analysis, data analysis, content curation, knowledge curation, all of those things that are very foundational. But I think I've told, I told you the story about the guy who said, oh, you don't need taxonomies. You don't need ontologies. You don't need metadata. I said, okay, what do you do with the data? Oh, you have to do data labeling. Well, data labeling is using metadata, and it's based on an ontology.
Daniel Cohen-Dumani [00:37:54]:
It's based on reference architecture anyway. But I think the critical thing now is, you know, how should, who should be educating executives? How should they be educated? And again, lots of vendors out there are offering these types of things, AI readiness assessments or AI strategic plans and so on. And the question is, if they're vendors, they have an agenda. There's some good sources out there. But what are your thoughts about how, you already mentioned getting your hands dirty and going in and using some of those programs? Any other thoughts about how executives need to be educated? Obviously, podcasts like this. Yeah.
Seth Earley [00:38:39]:
So there's obviously a need, and I will repeat this. Right. If you're an executive, you need to take it on. You have to become a savvy. I won't say an AI expert, but you have to understand the implication. You have to know what's out there. You have to be able to spell what an LLM is and explain what it does and what it doesn't specifically. Right.
Seth Earley [00:39:06]:
And you have to understand the pace of revolution and evolution.
Daniel Cohen-Dumani [00:39:12]:
Right.
Seth Earley [00:39:14]:
How do you do that? I think we talk about self experimentation, bring external folks. I know you do that. I've done that in my consulting world. There's plenty of folks. There's tons of online services that has short videos. But again, if you're an executive, you don't want to sit into a two hour video tutorial, but at the wait minutes, hey, this is understand the capability. Hey, did you know you could do that? Right? You know, Seth, can we have, you know, can, can AI create a podcast of you and I speaking? Absolutely. We write the script before then AI.
Seth Earley [00:39:53]:
And it will be there. Right. So can you create a nice presentation with AI? Absolutely. There's a million ways to do that now, right. So you have to understand what's capable today. And I always say your baseline is to understand what's available. Today is the worst it's ever going to be. As bad as it's ever going to be.
Seth Earley [00:40:18]:
Right. Which means it's only going to get better tomorrow. I said tomorrow. It could be next week, next year. So that's sort of your baseline. It's bad today. It's only going to get extremely. So much better that you better fasten your seatbelt and get ready for it.
Seth Earley [00:40:37]:
So listen to podcasts. There's a lot of very short podcasts. I listen to the AI breakdown, which is a five minutes a day Google AI breakdown. I think they rename it. It's the news of the day. It's often being reading pieces. There's a lot of mention. You can follow Ethan Malik, who's a Wharton professor.
Seth Earley [00:41:02]:
He speaks about AI in business sense for executive find a resource you like. I wouldn't say listen to 20 podcasts. Pick one you like. I like the short version, the 510 minutes a day. Right. I know my news of the day. This is what's new or what's coming or let's think about this. Some are very tactical.
Seth Earley [00:41:24]:
Here's an announcement of the week. Guess what? It's not a week without major announcement now and then get some help. Right. And it's not just you as an executive, it's your team. Right. Executive have to become extremely savvy, you know, great at understanding the impact down the road. So education is critical. I think strategy is important, Seth.
Seth Earley [00:41:48]:
But you know, you can look at it over a five year period. It has to be the next twelve months and you have to be ready to adjust it every three months. Right. Quickly.
Daniel Cohen-Dumani [00:41:58]:
Yep, that's it. Those are great points. And it really starts with the business problem that you're trying to solve. AI is a shiny bit of, and it's cool, but what are you really trying to accomplish with your business and what are you trying to impact in terms of a KPI and what are you instrumented for? So it really has to be tied to those things. And by the way, we'll put some of those resources in the show notes so you can send those URL's to us and then we'll include them. So just a quick shifting of gears. I just wanted to know a little bit about you. What do you, what do you do for fun.
Daniel Cohen-Dumani [00:42:38]:
What do you do outside of work? Tell us a little bit about.
Seth Earley [00:42:44]:
Yeah, yeah.
Daniel Cohen-Dumani [00:42:45]:
So, Daniel, outside of the professional, I.
Seth Earley [00:42:48]:
Mean, it's not technology, that's for sure. Right. You know, I enjoy being with my kids. I have four kids and my wife. Luckily, my kids are still in the DC area or even sort of graduating, walking. I love cooking. This is sort of my outlet of getting away from technology. It's something that hopefully technology is not replacing.
Seth Earley [00:43:13]:
It's very inspirational for me. Love to travel. My wife is a travel advisor, so we get to travel a ton.
Daniel Cohen-Dumani [00:43:23]:
I have to call her.
Seth Earley [00:43:24]:
Yeah, obviously, I go back to Switzerland a lot.
Daniel Cohen-Dumani [00:43:27]:
Yeah. Nice, nice. That's great. That's great. Well, thank you again for being on the podcast and sharing your insights. We appreciate everyone tuning in and listening, and we'll certainly catch you on the next episodes of early AI podcast. So, Daniel, again, thank you so much for your time and for your expertise and your insights.
Seth Earley [00:43:57]:
Thank you, Sef. It was so great to catch up with you, and I appreciate being on the show.
Daniel Cohen-Dumani [00:44:02]:
Yeah, yeah. And again, thank you. For those who've tuned in, thanks to the audience. If you learned something today or you enjoyed this, tell somebody about the podcast. And again, thank you, Daniel. It's been a pleasure.
Seth Earley [00:44:17]:
My pleasure. Thank you, Seth.
Daniel Cohen-Dumani [00:44:19]:
Okay, until next time, we'll see you all on the next early AI podcast. Bye now.