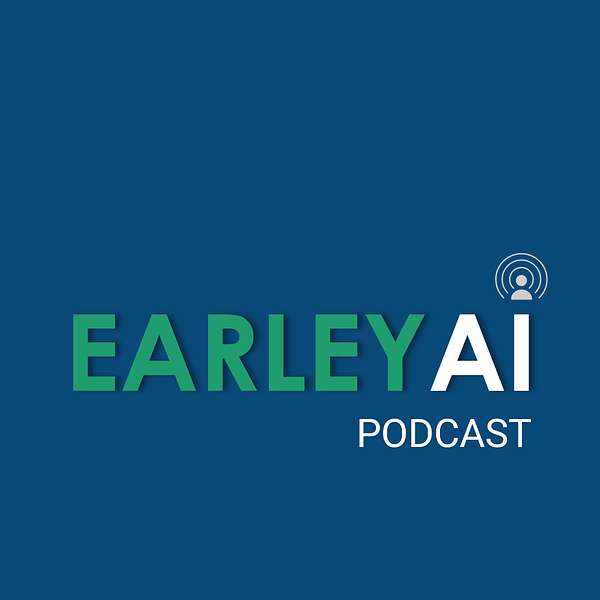
Earley AI Podcast
Earley AI Podcast
The Earley AI Podcast with Seth Earley - Episode #51 -Transforming the Workplace: Insights from Jason Radisson on AI and Gig Economy
This episode of the Earley AI Podcasts features Jason Radisson, an expert in digital transformations who has worked with renowned companies such as McKinsey, eBay, and Uber.
Tune in as Jason shares his insights on the misconceptions surrounding AI startups and the importance of having a quality team with enterprise experience. He also highlights the agility of startups in implementing new features and integrations, challenging the notion of slow processes.
Key takeaways:
-AI startups benefit from having a quality team with enterprise experience, allowing them to be agile and quickly implement new features and integrations.
- Non-tech companies often struggle to adapt to automation due to cultural barriers and legacy thinking, despite automation not requiring lengthy change management processes.
- The gig economy presents challenges in optimizing large workforces, requiring a balance between employer and employee perspectives to create win-win solutions.
- Organizations need to actively seek out innovative strategies and technologies to stay competitive, rather than relying on traditional approaches such as enterprise data warehouses and data lakes.
Quote from the show:
"The common approach of starting with an enterprise data warehouse and data lake is a fallacy. It's crucial to work backwards from customer-first use cases and focus on initiatives that will drive business value. By making quick developments and enabling additional investment, companies can harness the power of AI and machine learning technologies to transform their operations and stay ahead of the curve." - Jason Radisson
Links:
LinkedIn: https://www.linkedin.com/in/jason-radisson/
Website: https://www.movo.co
Ways to Tune In:
Earley AI Podcast: https://www.earley.com/earley-ai-podcast-home
Apple Podcast: https://podcasts.apple.com/podcast/id1586654770
Spotify: https://open.spotify.com/show/5nkcZvVYjHHj6wtBABqLbE?si=73cd5d5fc89f4781
iHeart Radio: https://www.iheart.com/podcast/269-earley-ai-podcast-87108370/
Stitcher: https://www.stitcher.com/show/earley-ai-podcast
Amazon Music: https://music.amazon.com/podcasts/18524b67-09cf-433f-82db-07b6213ad3ba/earley-ai-podcast
Buzzsprout: https://earleyai.buzzsprout.com/
Thanks to our sponsors:
Seth Earley [00:00:03]:
So welcome to today's podcast. We're really excited to introduce our guests for today. And we're going to talk about a lot of challenges and misconceptions around AI startups and AI startup capabilities, especially when it comes to large enterprises who may want to bring in an AI startup to help them with some of their challenges. We're going to talk about digitizing and managing the frontline workforce with AI and some lessons that have been learned from the gig economy. When you start thinking about all of the resources that need to be managed in a large city, when you talk about Uber or Lyft or other types of or doordash or other types of organizations where you have so much going on in the workforce, we'll talk about ways that gets applied to non gig economy types of organizations. We'll talk about user experience and how user experience is something that really makes a huge difference in terms of the ability to get AI successfully deployed in an organization and how critical that is to have that simpler user experience in order to take advantage of the capabilities that we're offering. So our guest today has successfully implemented and led digital transformations at companies like McKinsey, eBay and Uber. He's very passionate about leveraging technology in developing countries and empowering workers in the gig economy.
Seth Earley [00:01:34]:
Currently, he's spearheading a company that is focused on digitizing the frontline workforce to maximize productivity and efficiency. Jason Radisson, welcome to the show, Seth.
Jason Radisson [00:01:46]:
Thanks for having me on. Thanks for the intro.
Seth Earley [00:01:50]:
One of the things we like to begin with is understanding some of the misconceptions in the space, especially the way you're working in organizations and you're looking at how to develop and deploy AI. A lot of large organizations, number one, they sometimes underestimate the agility that an AI startup can bring to the table. The other piece of this is there's a lot of challenges around using startups because many times they don't necessarily have a deep track record and some of them can come and go. So why don't you talk a little bit about how organizations think about what are some of the misconceptions about how they look at AI startups and how they think about those challenges and how they can bring value to the organization?
Jason Radisson [00:02:41]:
Yeah, absolutely. Yeah, there are a couple of big ones. I think if you start from the top, one of the most important things, obviously, I'll make the obvious point first, which is got to be quality. It's got to be a quality team. Got to be a team that has worked with enterprises before. It doesn't need to be for 20 or 30 years, can be for two or three years. But rarely are you going to find a startup that is a couple of guys in a garage that have never seen the inside of a Fortune 500 company that are just going to come in and rock your socks. I think if you get beyond that and you're working with a good team, I think a lot of the corporate misconceptions, people just don't realize how quickly we work.
Jason Radisson [00:03:26]:
They don't realize that in a small team with experienced engineers who have worked at all kinds of scales and all kinds of different problems, we can literally have a feature in a week or two, a completely new feature. If it's something as simple as an extra filter, a tweak, an adjustment, a quick integration, people talk about, oh my God, APIs, and how are we going to integrate this stuff from a quick moving startup that's an experienced team? We can get stuff done literally in a week or two. An integration might actually literally be some senior engineers time, like 2 hours if that thing is well specced out. And most of that will be QA ing the 15 minutes of code that he had to write or they had to write to get that done. So I think there's a big misconception there. And probably the third misconception is a lot of companies just don't know how to buy services from a startup. They don't know how to interface with them. It's not sometimes rfps, the startup needs the feedback and sort of needs the close working relationship to be able to do things on the fly in real time and get them out and operating so that we can adjust to what that big company needs.
Jason Radisson [00:04:48]:
It's not an RFP thing. We're not sitting on top of 20 year old code, and we're going to negotiate around some terms and spend three months doing that. All of that is a huge waste of time and value for the big enterprise. So those would be three just kind of right off the top. But there's a lot that we see out there where we just go, oh my God, this is so difficult. And then there are, there are Fortune 500, there are Fortune 100 companies that know exactly how to buy from startups. Two weeks after you're talking with them, you're already rolling features out in some generally well protected, sort of low risk area of the business, but you're already out there operating together. But that's sort of a top five or 10% of enterprise companies, and the rest really, really struggle working with a.
Chris Featherstone [00:05:44]:
Startup, you know what, I'm a super fan of agility. I've had a couple startups myself. The thing that's interesting, and you've seen this probably, and I'd love to get your take, is when you walk into a, an enterprise organization and they say things like, well, we don't know if we can take on that much change that quickly. Right, which is actually, I get it, it's a real fear, but how do you kind of get around that or help them?
Jason Radisson [00:06:13]:
Yeah, oh yeah, absolutely. And I think what is so just sort of paradigm shifting such a different world that we're in now is really true. Automation doesn't require much change management because it's usually obviating work, which is very different from the ERP era, where we're mapping the company's processes and we're adjusting them, we're optimizing them, maybe ten or 20%. And all of that has to flow in this big, long, year long change management. All that stuff is pretty obsolete when you're talking true machine learning, heavily automated systems, it's literally plug in the system and then turn off the team that used to do that work. I think enterprise non tech companies just don't, they're not used to that kind of change management. I think it's very different. I would say the Silicon Valley experience, the big tech experience, um, is like, things work that way, it's the opposite.
Jason Radisson [00:07:28]:
You have this cultural pressure, uh, and I'll sort of paraphrase what happens a lot in, in Silicon Valley, but you know, basically your manager comes to you and says, hey, you know, Chris, you've been doing that thing three times now. Why haven't you automated it? Why isn't engineering here like automating? Like, what are you guys doing with, you know, 30 guys working on something manually? That makes no sense. So theres this bias in big tech to completely automate anything that is a stable process. Corporate America, typical large enterprise company, doesnt have that bias, doesnt have those muscles. Then you get to this. Machine learning is now, I think, getting more, at least attention, and we have this potential to do even more automation in white collar work than we would have if we didn't have Genai just given the interest levels, you look at all that and you go, well, there's a whole generation of corporate leaders in corporate America that don't know how to automate away their processes and start with, yeah, that's a year long change management. No it's not. It's actually stand up.
Jason Radisson [00:08:46]:
The automated process and then turn off the manual process. So very different world that we're in now.
Chris Featherstone [00:08:52]:
I think we get into this thinking too, of, you know, like, for instance, going to, like you said, a non tech enterprise and their best thinkers are still within the weeds of their own cultural barriers and dynamics and everything else and legacy thinking. And so they're the ones that are, quote unquote, supposed to be the tip of the spear. And yet, to your point, you know, they're, they come in and say, no, no, no, that's going to take, like you said, a year to actually really get implemented. And we have to do, like, if we want machine learning, we have to collect all of our data at the same time and figure out how to get it into one spot, which is not the case, you know, totally.
Jason Radisson [00:09:31]:
It's one of the. Yeah, yeah. I couldn't agree more. I think it's one of the biggest fallacies in it is that everything starts with an enterprise data warehouse and a data lake. And it's nonsensical, it's very expensive, it's very self serving for database vendors. They've trained a whole generation of it leaders to think that. But it's ridiculous. You have to work backwards from the use cases that ring the cash register.
Jason Radisson [00:10:05]:
Those may require you to instrument your mobile app with two or three new variables, and that might be 80% of the value from your database. You need to, I think, always have that customer first use case first mentality before you do any investments. We just see it. We see it time and time again. There are entire very large cap database vendors out there that have built their whole sort of legacy on that approach. Yeah, very, very different world. I guess I could see there was a time where some of that stuff was relevant because databases were so, they were so expensive and so it was a big capex project if you were going to think about how you were going to collect any data. If I think back to the wireless industry 25, 30 years ago, it was an enterprise project to get a little database together.
Jason Radisson [00:11:07]:
But these days, that's a laptop, not a hundred million dollar item. And it's just crazy to see what companies go through and how twisted up they get and often hiring in our new digital leader and that person comes in and spends three years building a data warehouse before they even really have any use cases that are working.
Chris Featherstone [00:11:32]:
To be, to be fair, like that's. I mean, Waterfall had its time too, where it actually was relevant, but also also is synonymous with a huge capex type of approach as opposed to fail fast. With cloud based services or whatever that is.
Jason Radisson [00:11:47]:
Right. That are super. Yeah. And I think it's just, it's fundamental to the startup approach. And I think the reason big tech is so different from non tech enterprise is just, you know, they were all startups at one point. They all know there's no way Facebook is going to forget how to do some iterative stuff to see how a product works, given the DNA that they're built on.
Seth Earley [00:12:14]:
Quick question. When you start going through these enterprises, and you've been working in both startups and large corporations, what challenges are there right now in educating executives? They moved through part of the learning curve, but I think there's still this kind of, well, AI is important. They're still not completely clear on what to do with it. They're definitely not clear on what they need to do to make it work successfully. Where are you, where do you see the challenges in breaking through the noise and educating executives?
Jason Radisson [00:12:50]:
Yeah. Yeah, definitely. I mean, I think I kind of break those challenges down into two buckets. I think there's, there's a group of companies that has a burning platform, and so AI, whether it's Genai or whether it's some machine learning and more specific systems technology, is a way to sort of fix that big, big problem or that big opportunity, get to that big opportunity that they all know about. And then I think you've got a whole class of companies where they might even have existential risk around the corner. They're not really looking at it. They might not have done the far out planning to put those pieces together to see shoot in two years, actually, we're going to get a whole class of competitors because of this technology. They may have a lot of internal, they might have five or six items that are occupying everybody's headspace.
Jason Radisson [00:13:49]:
And so they can't really get consensus momentum around which one to action on first. So I think you just, I don't know, it's difficult. I think you kind of have to think about what are your actionable pivot points in all of this? And as a corporate leader, where do I recognize, how do I recognize that I've got a big opportunity and then how do I execute on it? I think those are the two, right? The one is sort of the strategic reckoning. The other is like actual muscle to go spin something up. And I think there's a good best practice out there, which is like, it's basically the six week execution practice, which is like, if you have a pivot point, try to do something about it in six weeks. If you. It just sort of, it's enough time to get some rough product together with five or ten folks in a small engineering team. It's not a big corporate investment if you have to get some outside help from an engineering shop to go do that, whatever the case, but making sure that you're actually doing things and you're not just talking about it and going to meetings for a year and a half, vaguely keeping this stuff in mind, but rather actually doing something.
Seth Earley [00:15:13]:
I think you hit the nail on the head when you said what's going to ring the cash register? What's going to improve. Yeah. Efficiencies, what's going to have an impact? And that's through understanding those processes and those use cases, saying, here's our objective and then what's going to enable that objective and then how do we measure it? What are our baselines? Right. And how do we measure intervention that's going to enable that.
Chris Featherstone [00:15:37]:
The key, though, was definitely from the cash back because that's the value prop. But I think the critical notion of that too, for all of us that are in this spot, is working backwards from that goal and just in terms of that perspective, because otherwise it'll just go on and conflate forever and ever and ever, instead of, hey, listen, here's exactly what we're driving at, and we've got to be able to do that. The beauty of that too, is we've done it in a week or we could do it in six weeks, whatever. The true goal is to see that one quick thing that's going to help with the additional investment.
Jason Radisson [00:16:10]:
Yeah. And that gets you to these hard questions, the make versus buy question, the technologically possible. Right. You might come into some hardware or software constraint, like, look like the hardware that's available today doesn't actually support that use case. Let's keep our eye on the companies that are developing new stuff in that hardware dimension, whatever the case might be, but it actually gets you on top of the pivot point rather than just, like I said, vaguely knowing the risk is there, but not being able to execute on it.
Seth Earley [00:16:44]:
Yeah, yeah. And I want to ask you about how you've taken. We didn't mention exactly what you're doing today, so I want you to kind of go back and talk about what you have done with other organizations in terms of managing the workforce and managing the gig economy types of workforces, and then how is that kind of brought you into this space that you're working in today and what are the lessons learned? So you worked for Uber for a while. Do you want to talk a little bit about that work?
Jason Radisson [00:17:20]:
Yeah. So there's a lot, I ran a region in the US in the early days of Uber, and there's a lot to that that's not specifically technology related. There's a lot of government relations and otherwise there. But what I would say specific and germane to what I'm doing today and the kinds of problems the team and I are working on, what I realized from the gig economy was if you take the software as it is, and essentially a marketplace for getting work done, a two sided marketplace. In the case of Uber, in the case of some of the delivery apps that I've worked with, invested in a three sided marketplace, there's a ton of efficiency there. And so broadly speaking, what I was thinking about in founding Movo was how can we bring the kind of efficiency that we see in the gig economy to corporate America, to large foreign multinational companies that might be out there with tens of thousands of workers with a ton of inefficiency? And very broadly speaking, how do we improve the lot of the frontline worker, wherever they might be, whatever inefficiency and difficulty they have in getting ahead? So I think if you look at it as a win win situation, and I think of platforms and marketplaces as, you got to make them win win if theyre going to be sustainable and if theyre going to be, you know, profitable and not just a big leaky bucket that eventually people forget about a couple years later, you look at the problem set that's out there and a couple of areas of technology become really evident. And I'll preface this all by saying it's all machine learning. I mean, it's, you look at the core of the different features and functionalities and systems.
Jason Radisson [00:19:20]:
They're all machine learning systems. So basically, you've got a massive scheduling capability. So one of the things these marketplaces do is they slot workers to demand for work extremely efficiently. The other thing that they do is they distribute tasks and they give you sort of a very transparent task completion, task issuance, and task completion set of technologies. And I looked at those and I thought, I, you look at the logistics industry in the US, you look at clinical staff in the US healthcare system, you look at housekeeping in the us hospitality market. You look at a lot of these big job classes that employ, by the way, millions and millions of Americans, and they're not digitized, and they're certainly not digitized in any kind of real time, streaming, optimized way. So that was sort of the thinking that got us to Mobo and the team and I started in 2019 with some experiments. 2020, we got very active in the beginning of the pandemic.
Jason Radisson [00:20:34]:
We all had just gone full time in January of 2020. And then in March of 2020, as the pandemic really hit and the shutdowns started happening, we actually closed a deal with Amazon and started helping them with some of their most burning labor issues and in particular in Minneapolis in everything that came with social unrest and everything that was going on in those early days of the pandemic. So a lot of learnings along the way. Along the way, we've hired 700,000 people in the US and deployed them. As we look at the latest iterations of our product, we're really extremely focused on the scheduling problem because I still believe that's one of the biggest drags on the economy. But that's movo in a nutshell. And the kind of services that we're out there providing.
Seth Earley [00:21:25]:
And it's Movo co and you're founder and CEO, which I didn't say.
Jason Radisson [00:21:35]:
Sorry about that.
Seth Earley [00:21:37]:
But it's interesting when you think about what's going on with the gig economy, and you have thousands and tens of thousands of workers that are out there in real time challenges. And you kind of made the analogy to battlefield conditions because everything is changing. You're deploying assets, you're redeploying assets, you're having problems, things are happening, and then you still have edge cases that humans need to deal with. But you're taking the brunt of all of that brute force work, of aligning supply with demand and lining up tasks with workers and making sure that those are completely fulfilled and all of the follow up and all of that stuff that happens with it. And I can see how that could obviously be applied to large workforces and at multiple levels, going beyond scheduling, trying to corral and understand what all of these remote workers are doing and how their work is being accomplished and how they're using automated tools. And it's kind of like this expanding universe of capabilities that you could be looking at and thinking about in terms of how do you optimize tens of thousands, hundreds of thousands, millions of workers in terms of what they're doing and how they're contributing to the organization. So it's a fascinating space. And one of the things that we had talked about is the fact that there's an organization, there are other organizations that do this stuff, especially with the scheduling apps.
Seth Earley [00:23:11]:
However, one of the things, and at the core, you could say function by function, feature by feature, maybe they're equivalent or whatever it is. But the big difference is that user experience. So when you start thinking about how do people use these things and how did, because we've all been in terrible applications, and what is it about a terrible application that makes it terrible? It doesn't align with your mental model. It doesn't align with your thinking about how to solve that problem and how to go about your task. Why don't you talk a little bit about your view of user experience when it comes to the machine learning and AI?
Jason Radisson [00:23:51]:
Very much so. Broadly speaking, a lot of people are working on what I would broadly call the problem of who should work where. And you can look at whether it's an ETS or a hiring platform, some of the job boards, like the indeed of the world, people are working on who fits this particular job, matching that supply and demand. What we've done at mobile is we've taken it about ten steps further to where should this particular person with this particular skillset be working in this instant? Based on everything we know about them, based on what we know about their scheduling preferences, based on what we know about their employer's demand for labor, in this moment, we integrate in real time with the labor forecast and some clients, we help them build their labor forecast, and that gives us a user experience because of that real time machine learning, optimal allocation of each person's minute of work, we're in this position to give a very, very modern worker experience. So the worker, the employee, any given time, has a ton of opportunity, at least the opportunity that exists in the company to go pick up an extra shift, to pick up an extra credential, to be able to work a different shift or to be able to level up. All of those opportunities are completely transparent to the worker. Mostly, if they're interacting, they're just interacting with the mobile app. They're not having to go the old school way of, you know, having lunch with supervisors to try to figure out who might have an opportunity.
Jason Radisson [00:25:38]:
You know, supervisors don't have to go the old school way of calling and trying to find if you don't have the text, the mobile numbers of the different workers that are out there. All of this is just handled by bots. The bots make sure that everybody's in the right spot, and the bots surface all of the opportunities that are out there in the company. So it's a long slog to get all the machine learning in place, to be able to do that. But once you have that kind of capability. It's such a modern, light, easy experience for the employee that nobody wants to go back. And so I look at it as in some ways, solving scheduling is one of the best things you can do for worker engagement, for giving them the feeling of empowerment. They're more empowered over their own time and flexibility.
Jason Radisson [00:26:32]:
They're more empowered over their career path than almost anything else you could do.
Chris Featherstone [00:26:37]:
I'd love to get you build workforce management systems for call centers. And I've always loved the gig economy perspective of this. Like, hey, I want to self choose. What have you seen, though, is the inverse impact of that on the psychology of almost entire borderline entitlement slash empowerment? Does that make sense when we're thinking about those, especially with an organization that's coming from years, like decades or centuries of culture that is very much steeped in work for 40 years or whatever? I'd love to get a take for what you've seen psychology change in that.
Jason Radisson [00:27:17]:
Oh, totally. And, you know, and I'm not sure how many HR leaders might be in your audience as well. I think it's very relevant for them also. Look, I think there are guardrails. Most people focus on the guardrails. The guardrail, on the one hand, is the employer guardrail, and it says, this is the schedule, take it or leave it. I think the other guardrail is sort of the completely gigafied, hey, this is when I'm going to work this week, take it or leave it. Employee perspective.
Jason Radisson [00:27:45]:
The way we solve it with most clients is we will fill the schedule based on your preferences. However, if we don't have anybody that wants to work a shift, we're going to put somebody in. It's somewhere in the middle. We're meeting employees somewhere in the middle. And like I said, I think success long term of any workforce, really any marketplace, but any workforce is, it's got to be win win. And you have to kind of find these kinds of solutions. And I think we've got it now. That doesn't mean that we don't have employers that see it a little bit more on their side.
Jason Radisson [00:28:28]:
And we're still figuring it out on a case by case basis, but it's basically that. And it starts with the employee experience, starts with the employee putting their preferences in that then mostly get honored. The other thing, too, we automatically do a bunch of substitutions. We do substitutions. We do peer to peer shift swapping. So even if you don't really get your preferences in your main shift, your main shift is nine to five. Monday to Friday. But you would really like to have Thursdays off.
Jason Radisson [00:29:00]:
But the company says, too bad, sorry, we can't honor that because we need you on Thursday. Right. You can still put in to be an alternate on a bunch of other shifts. Say you want to pick up some weekend hours and you get those. So even if we can't always honor, 100%, your preferences, we might be able to open up some new space and some new earning potential that you wouldn't have been able to get otherwise. So it's not just black and white. No, on Thursday, but more case by case. And I think that's just life.
Jason Radisson [00:29:36]:
And I think the best supervisors have kind of always done that. We just. In creating the system in the way we have, we've been able to replicate that in this automated way.
Seth Earley [00:29:46]:
It was always fine on Thursdays, we won't say no.
Jason Radisson [00:29:49]:
Right, right. All right. And maybe one Thursday is slow, so, you know, maybe you get a Thursday a month off, you don't get all of them off. So to us, that feels modern. And I think it. We've been talking, I mean, it's a compromise. I think we're all sort of dealing with generational shift, right? We go to x to z, to X, Y and z, and people have different expectations. And look, it is not going to be 100% the employee guardrail.
Jason Radisson [00:30:18]:
Nobody can run a business that way either.
Seth Earley [00:30:20]:
Of course.
Jason Radisson [00:30:21]:
It just is what it is. I think at this point we found.
Chris Featherstone [00:30:24]:
Too, some of the, we'll say older generations were able to leapfrog into a gig economy thinking because they had been out of the workforce for so long, that they kind of skipped that generation of, oh, they used to be super, you know, like cultural, nine to five, and then they were out of the workforce for a while. Now they jump back in, and now it's this perspective of complete flexibility in theory. But, yeah, it's been really interesting. So, yeah, that was the hardest binary problem to solve in a lot of cases was, here's the plan. However, when the plan hits reality and you have people that are out sick, and then Corona provided a whole new perspective. Just the binary goes out the door quick around, like, oh, it has to be flexibility. And there's really a. I think there's.
Jason Radisson [00:31:17]:
Some really interesting, and as you guys know, I've worked in China, and in particular with Didi, who bought my last company. I've also worked a lot in Latin America, and I think there's a lot of interesting stuff coming out of the developing world. Large companies in Latin America see the gig economy as the competition for labor they go. If you're running a bottling plant, you're a massive bottling plant. You're competing with rapi and Uber for employees and all the employees that are coming in, all your front line is used to having everything be based in a mobile app and having that complete flexibility. So I like to think that with the solutions that we're finding with each employer and sort of where that slider is, that we're actually providing an even better experience. Because the flip side of the gig economy is you have perfect flexibility to pick whatever hours you want. But you may pick really crappy hours.
Jason Radisson [00:32:17]:
You may pick an hour where there's a schedule where there's no riders or there's no orders to be delivered, and so you make no money versus if a hospital is having you come in, it's because they have work for you. It's not like you're not going to get paid in our world.
Chris Featherstone [00:32:34]:
That's awesome.
Seth Earley [00:32:36]:
I have a question about organizations taking risks and looking at where they are in terms of their AI journey. One of the things we talked about in our prep call was that you'll talk to some executives and they'll say, well, we're getting our infrastructure together and our tech stack and we're doing these things and we're not ready to do that. And I hear that a lot. We're not ready. We're still trying to figure out what the bet is. You had mentioned the fact that there can be million dollar bets and there can be billion dollar bets. I think you mentioned in terms of the implication for organization. And even if we say, even if we bring it down in order of magnitude or two and say $100,000 bets versus million or 10 million, you need a couple of different things in order to do that.
Seth Earley [00:33:31]:
Number one, you need an executive that believes in it and that has enough social capital to be able to affect change. Obviously, you need budget for that. You need the right set of use cases, but you really do need that kind of expansive thinking about where the organization can be and what it can do. And do you want to talk a little bit about that mindset and what you've experienced? And I think it was really interesting the way you said multimillion versus multibillion, or even, again, if you say 100,000 versus million, but talk a little bit about that mindset and how organizations need to cultivate that and executives, how executives need to go into these things. Thinking about potential impact.
Jason Radisson [00:34:16]:
Totally. Well, to try to put it a little bit in my answer, a little bit as a pivot point, I think the pivot point is if you're in a company and there is a lack of a common understanding of what the big automation opportunities are. So my challenge to anybody in a large corporate environment would be, can you name off the top of your head four or five big automation opportunities and put dollar opportunity like dollars behind them. Like we were saying, this is a $2 billion opportunity, this is a $20 million opportunity, this is a $200,000 opportunity, because I think that's the place, the mindset, the headspace you have to be in. And then everything just becomes execution. And in terms of change management, yes, you're going to have to figure out the path that your company and culture and everything demand to get there. But at least you're clear on the what. I think it usually starts with.
Jason Radisson [00:35:29]:
Well, the lack of even understanding the what. One of the biggest examples that takeaways and examples from my career. So I worked for Gary Loveman at Harrah's entertainment and then Caesars Entertainment. I was the post merger guy. I ran database marketing there, and the statistics and algorithms team and post merger management. And we, when we bought Caesars, part of the justification were both publicly traded companies. We had literally twice the EBItDa per square foot in our company that they did. And the reason we had it was largely due to an algorithmic approach to base management.
Jason Radisson [00:36:10]:
Like, we would get a new customer, someone would come into our casino for 5 seconds, and we would figure out what they were worth, and then we would start disproportionately spending on them, on the customers who were worth it, who had the upside. And we did that just famously. There's a bunch of HBS case studies out there, and our business review articles and other business press and academic work that's been done around that. But the crazy thing was, it was all published. Literally, our algorithms were published for years and years, and literally, Caesars Entertainment, right across the strip from us, didn't employ any of the strategies.
Seth Earley [00:36:51]:
I, you know, that mind boggling. Go ahead.
Jason Radisson [00:36:55]:
That's sort of, that stuck with me my entire career, where I say one of the biggest problems in corporate America is a lack of sort of understanding where the big levers are. And it's not an individual thing. It's not like there's nobody at Caesars that ever understood that. But it was sort of an enterprise level thing that the company couldn't sort of click the right pieces into place in order to execute on the playbooks that were winning in the industry.
Seth Earley [00:37:28]:
So this is where I famously say, at least famously to myself, that having the answer doesn't matter. Right? Like I said, having the answer doesn't matter. Like, I can publish articles and say, this is the way it is, and here's how you do it, and here's the case study. And yet there's this, you know, there's this weird, like, gap. And I'm so glad to hear you tell me this, right. Because it makes me feel like it's not just me that you had published these things. They showed the value. HBR picked it up, all of these.
Seth Earley [00:38:04]:
And, I mean, I would want to go back and interview those executives and say, what were you thinking? Or what were you, like, why? Why did this, did you not know about this? Like, had you heard about this? You know, I mean, I'm sure they have.
Jason Radisson [00:38:19]:
Right, right.
Seth Earley [00:38:20]:
Has anybody done any kind of forensic discovery or research?
Jason Radisson [00:38:24]:
I mean, you know, do it? Yeah, yeah, totally, totally. It's so long ago. But I can. I can talk a little bit about, you know, sort of the experience in the post merger. You know, clean room, but then, or non clean room, but then sort of the post merger stuff. But what I would say, take the example.
Seth Earley [00:38:41]:
When you say clean room in that context, you're meaning regarding data or what do you. How are you using clean room?
Jason Radisson [00:38:47]:
What I mean is in the pre merger phase where the two companies plan together.
Seth Earley [00:38:57]:
Right.
Jason Radisson [00:38:58]:
And so we got a good look into things, and I'm not like, this is like, the companies have changed owners numerous times, and there have been other books written about. This is all public information, everything that's happened. Yeah, but let's take the example, in an algorithmic example, let's take yield management. So the pricing of rooms. So there's, for those that are out in the hospitality world, there's an old school way of doing it, which is, there's literally a guy or a gal who prices rooms, right? And it's the mom and pop way. And it's like, here's our calendar, and here's what our rooms cost. And that was replaced kind of famously, kind of with some technology that came out of commodities trading back in the eighties and with american airlines and with, like, the real time pricing of airline seats. And then the casino industry deployed that through.
Jason Radisson [00:39:52]:
There was this company called prose that was running around sort of selling real time room pricing. And at Harrah's entertainment, we had nine segments, and we priced for rooms in real time. And we had kind of done this. And this was sort of a top down decision. I think Gary Loveman sort of came to the company. If you looked at Caesars Entertainment, they had manual, old school room pricing pretty much everywhere in the company. And they were working on an extremely complex 99 segment room pricing system that hadn't really been deployed. The problem with 99 segments is you don't have enough traffic.
Jason Radisson [00:40:40]:
You just get into very messy answers with very low statistical significance. We'd long sort of rejected sort of a extremely fine brain model. Nine segments was enough for us. But, I mean, we can talk about other things that they kind of got wrong, but, like, fundamentally, like, they sort of made. And, you know, like I said, this is all these people, all the roles have changed. Everything's changed. This is 20 years ago, 20 plus years ago. But I think the important learning is, like, they made a essentially sort of a extremely half hearted effort to even sort of pay lip service to what needed to have happen.
Jason Radisson [00:41:24]:
And that, I think, is where a lot of this stuff gets bogged down. It's like, even if the strategy, even if there's pressure to have the strategy in place, have you, and what should they have done? They should have taken our model and exactly replicated it in one casino and proven the difference, proven that they could get the two x lift in revenues per room and EBITDA per room, and then had the momentum to be able to have done more change. It might have been a very different sort of history of the industry if they had done even that simple thing. So it's a long story, but the moral is really short, which is you've got to, I think you've got to know the what. But to your point, knowing the what.
Seth Earley [00:42:13]:
Is, uh, audio there, Jason, can you hear?
Jason Radisson [00:42:17]:
I can hear you. Yeah.
Chris Featherstone [00:42:19]:
Yeah.
Jason Radisson [00:42:21]:
Okay. Huh?
Seth Earley [00:42:23]:
Yeah, it sounds like I've lost audio.
Chris Featherstone [00:42:25]:
You know, I think.
Seth Earley [00:42:27]:
Lost your audio. Here we go. You're back. Okay. Don't know. Yeah, we are. Sorry.
Jason Radisson [00:42:33]:
Yeah.
Chris Featherstone [00:42:33]:
As, as they say, you know, from the old, you know, from what you were saying before, you miss 100% of the things you're not looking for. And I think that's what happens, is you get these organizations get in the weeds and can't figure out what the hell to do because they don't know what to look for it. And to your point, they don't have anybody that's there to say, hey, by the way, did you see what they're doing across the street? And is that interesting? Should we think about that? And usually that's lower below the budget line type of folks that are seeing that kind of stuff, but above the budget line. People think they've got it made in the shade, so they're doing it and.
Jason Radisson [00:43:09]:
They just mess in huge ways.
Seth Earley [00:43:12]:
I know we're getting to the end here. So, Jason, tell me a little bit more about your world. Do you have kids? Do you have hobbies? Do you have pets? Do you travel? Do you, what else do you do besides work?
Jason Radisson [00:43:25]:
Yeah. Family and outdoors are really the things that I do outside of work. I have four kids from 18 to three. We're busy on that front.
Seth Earley [00:43:37]:
Yeah.
Jason Radisson [00:43:38]:
And, you know, we're, we moved to Minnesota in the pandemic. Are you, are you able to hear? I'm not sure about the audience.
Seth Earley [00:43:45]:
I can hear you. Yeah. Okay.
Jason Radisson [00:43:47]:
Yeah. And we moved to Minnesota during the pandemic. And, you know, I've kind of grown up in the outdoors and do everything from skiing to fishing and hunting, and I'm just out in the woods with the kids as much as I possibly can be outside of work.
Seth Earley [00:44:05]:
So after the three year old, what's the next age? What's the next old age?
Jason Radisson [00:44:10]:
Four. Yeah. Yeah. People often think they're twins. Yeah. Yeah, exactly. We re upped. Yeah.
Jason Radisson [00:44:20]:
Yeah.
Seth Earley [00:44:22]:
This has been great. I've really enjoyed chatting. And what do you see as the next frontier? You know, when you talk about workforce management, like, I could see this being the beginning of a lot of stuff. Like, in terms of optimizing a workforce, what do you see in the horizon? What's the future look like?
Jason Radisson [00:44:40]:
Well, I mean, I think it's funny to watch the evolution of Genai, and I think you can't help but look at Genai and realize that it's a general technology that sort of needs to, to come back around to solving real specific use cases, and that evolution is going to happen. We saw it with machine learning. I think machine learning was a little bit stealthier, a little more under the radar, and just kind of done. There was just a day when we all woke up and Amazon was there with a real time optimized homepage. We didn't realize it. There was just a day we woke up and american airline was charging us a specific price for a seed. There are so many fully automated, very smart systems in the world. And I think what's going to happen with Jennai is it's just going to come back around and it'll enhance some of those engines.
Jason Radisson [00:45:44]:
It'll support a ton of new use cases. But I think that process is just in its infancy. I look at what that means for work. It probably means some of the same things that we've seen in machine learning companies, which is we need a lot more of these sort of operations research, industrial engineering job classes. I've been bullish on that university program, college program for, for 20 something years. I used to, speaking of Caesars, Harrahs lead campus recruiting for Undergrad and for business school and grad school. And those types of roles are as hot today as they ever were back then. Those are the people that are going to be leading these systems and thats where a lot of the job growth is going to be.
Jason Radisson [00:46:40]:
And im very bullish on the frontline workforce. I don't think we're going to be replacing nurses, home health care people, a lot of hospitality service people. Any of that is going anywhere anytime soon. So you look at all of these advancements, probably the role of supervising and we're going to see more remote plus forward deployed resources I think is just this new evolving model of work. And this is the new reality. We are half the time going to be talking to people and running teams on Zoom and other tools like mobile. There's going to be a little sliver of the old world, but I think we're already pretty much into this new mode and I'm just excited for everything that it needs.
Seth Earley [00:47:35]:
It's going to change quickly and it's Mov co and you can connect with Jason Radisson. R A D I s s o N. That will be in the show notes. I really want to thank you for joining us today. Jason, thank you so much for your time and your expertise.
Jason Radisson [00:47:57]:
Thank you guys. Seth, Chris, both for having me on the show. It's been a real pleasure chatting with you.
Seth Earley [00:48:02]:
Great to the audience for listening and tuning in. This has been another episode of the early AI podcast. And thank you for Carolyn, for her behind the scenes work and Chris and again, thank you, Jason. We'll look forward to continuing our conversations and collaborations. I really enjoyed it today. Thank you.
Jason Radisson [00:48:23]:
Thank you.